Synthetic Data Types
Research Project
2023 -
This study utilizes large-scale-text-to-image (LLI) models to investigate possibilities to describe building types data-centric. With the introduction of "data-centric typologies" we hope to challenge traditional architectural classification systems while reviving type as an architectural strategy to link socio-economic contexts to the physical form of a place, building, or city. By examining AI-generated images of various city buildings, the research explores compositional characteristics, realism, and model limitations. We generated and segmented a synthetic dataset of 450,000 images into individual building segments, conducting a statistical analysis of compositional features across 5600 cities. With our first objective we ascertained whether LLI models can generate realistic portrayals of a wide array of local types, and to identify existing limitations. Secondly, we categorized the data to compositionally compare cities, which subsequently enabled us to identify key compositional metrics for this type of big-data analysis and visualize their hyper-dimensional feature space. Finally, we compared insights from the derived compositional score with established socio-economic city rankings. Despite dataset biases and limitations, our results indicate synthetic databases provide a deeper analytical basis than traditional methods. The generated dataset alone paints forensic landscapes of locales that are not typically showcased. Particularly from a pedagogical perspective, data-centric investigations can serve as a valuable tool for illustrating the diversity of cities and living modes. The findings show that socio-economic attributes like quality of life are more closely tied to neighborhoods or projects than entire cities. Consequently, architectural typologies are most effective at a human-oriented scale, interfacing city with architecture.
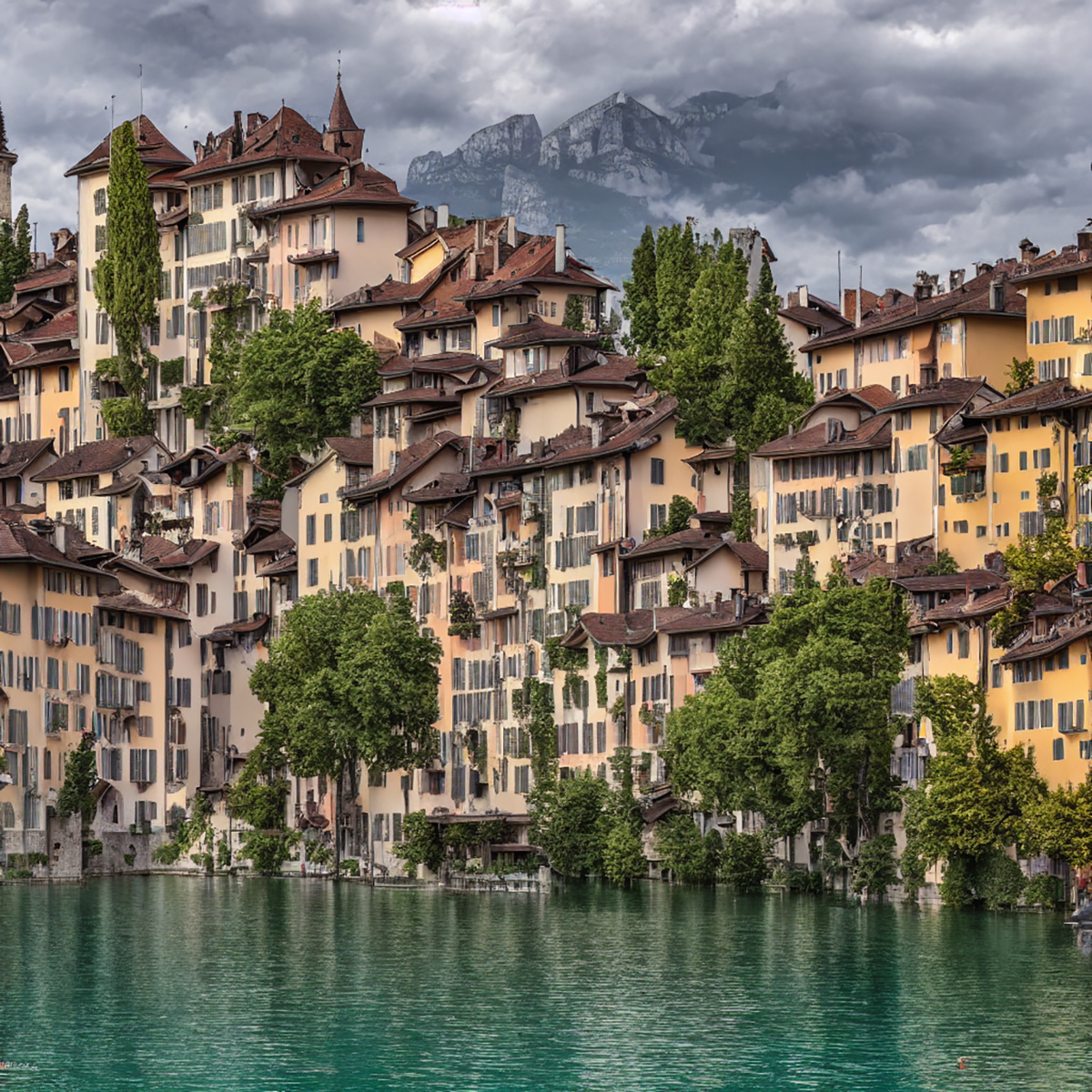
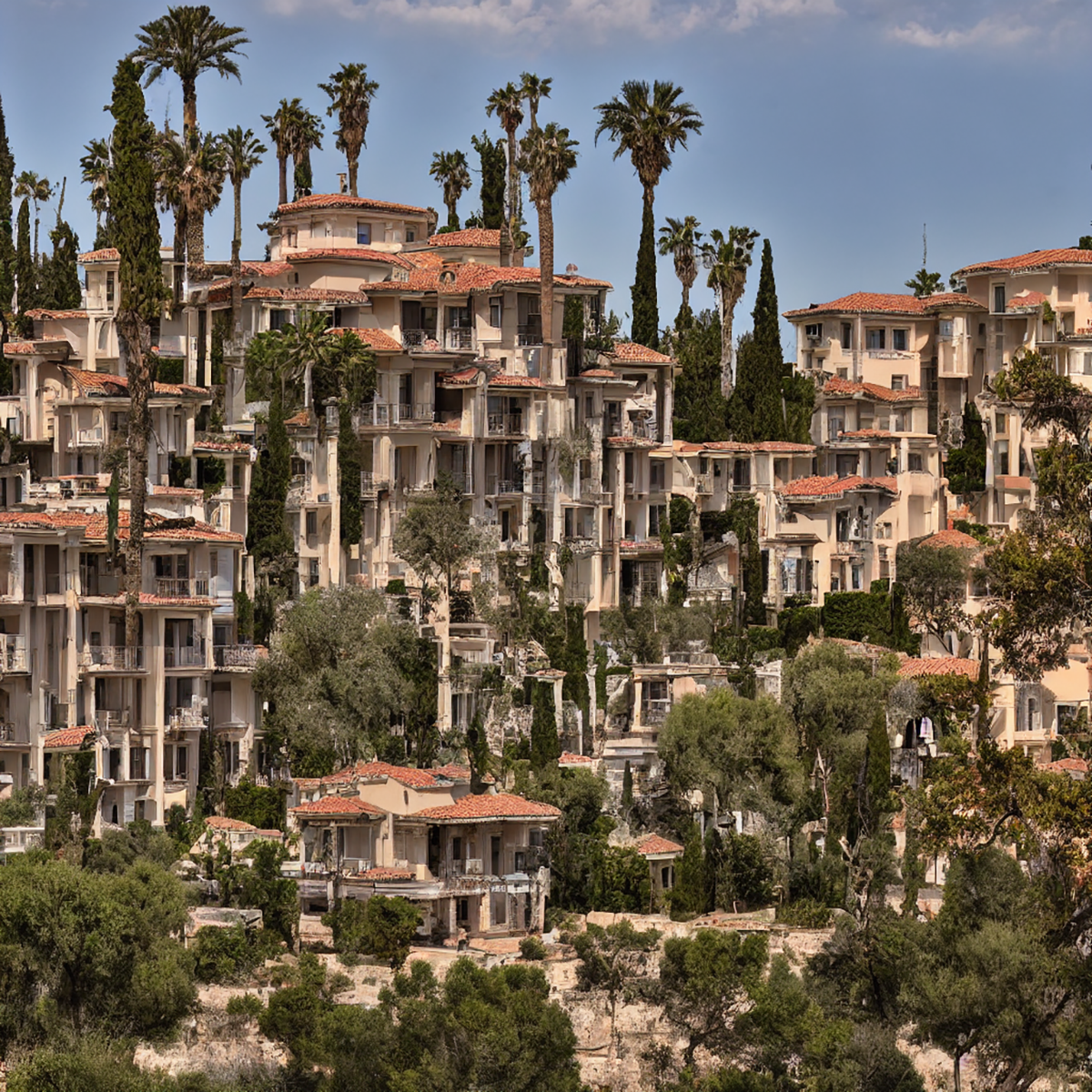
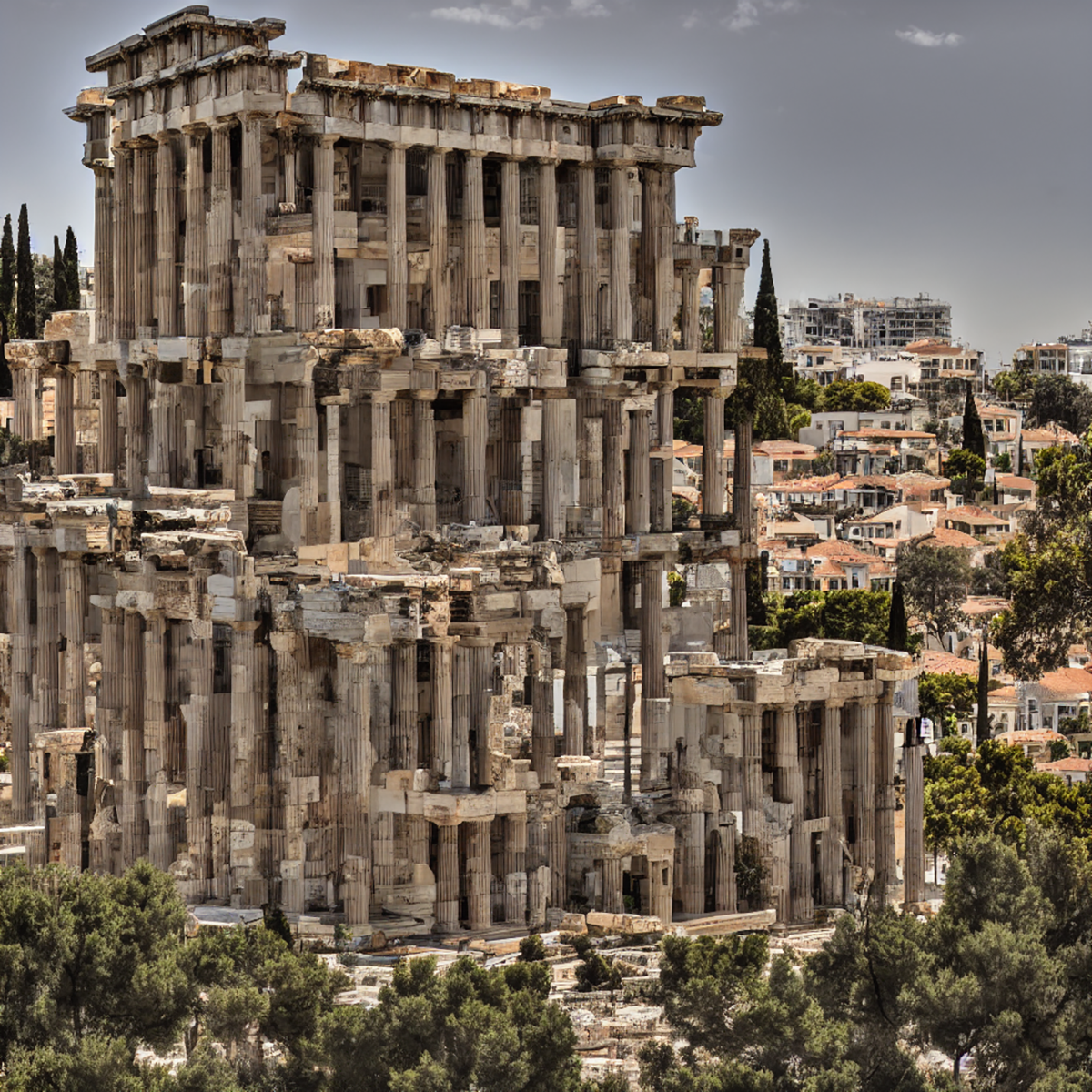
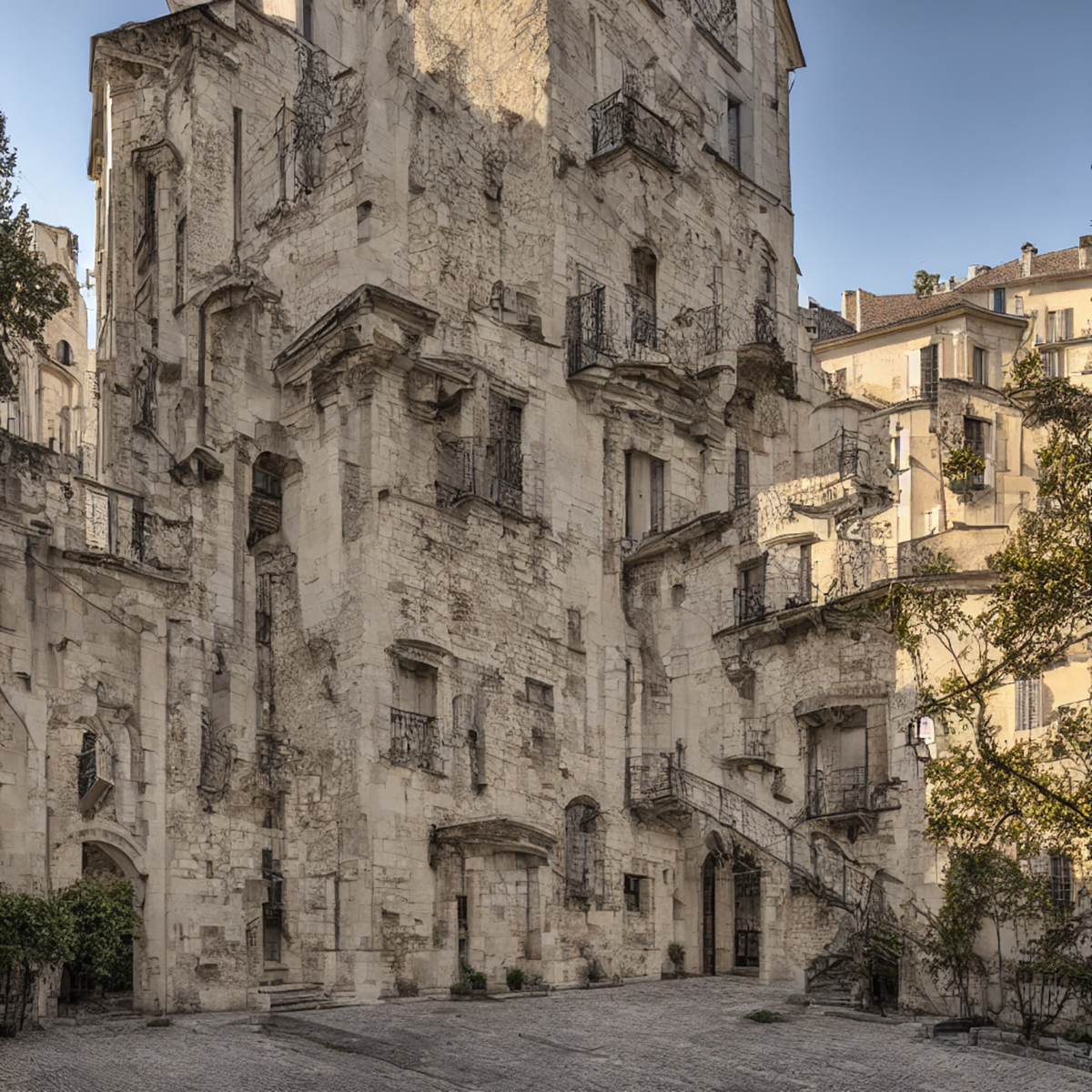

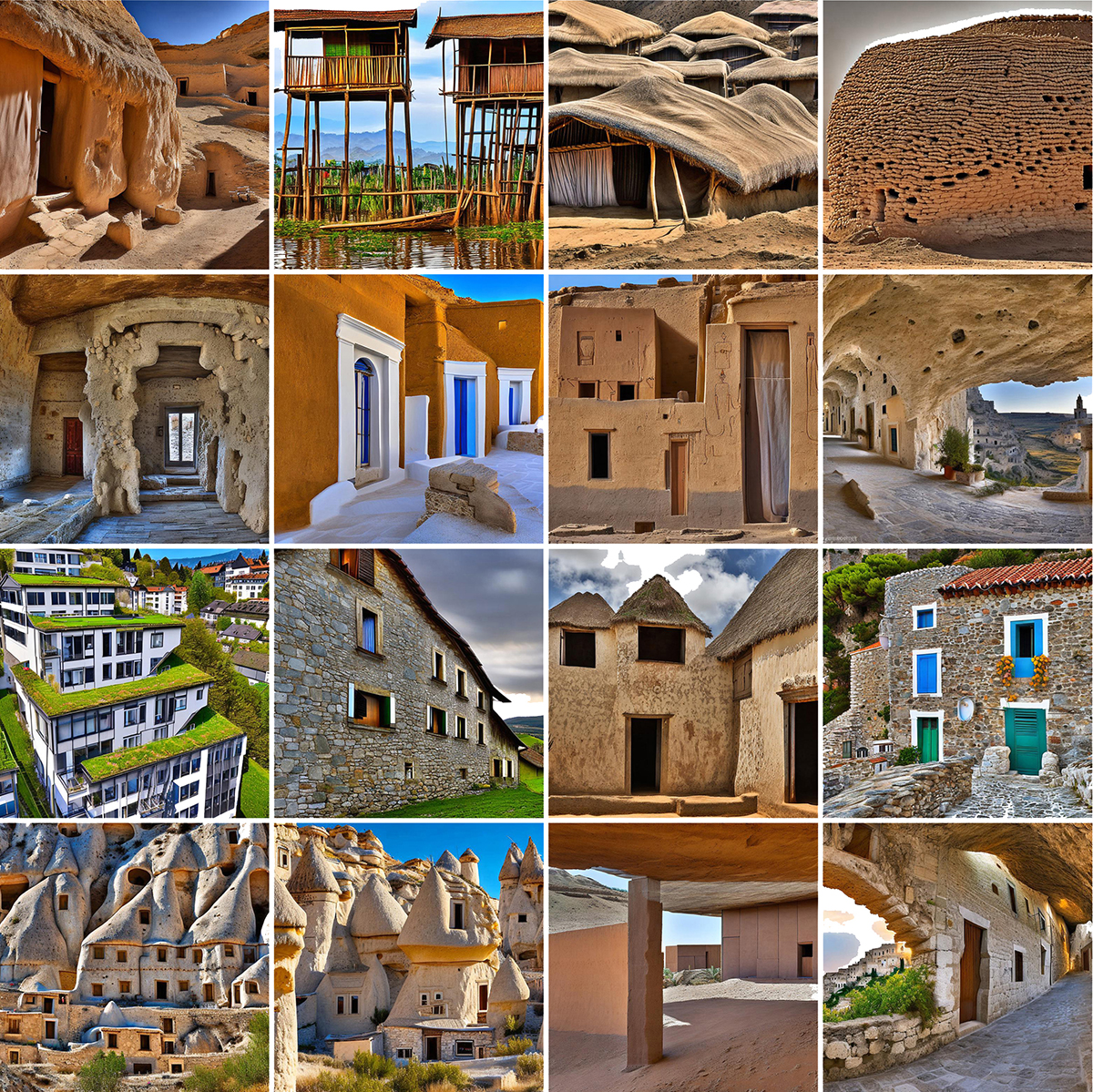
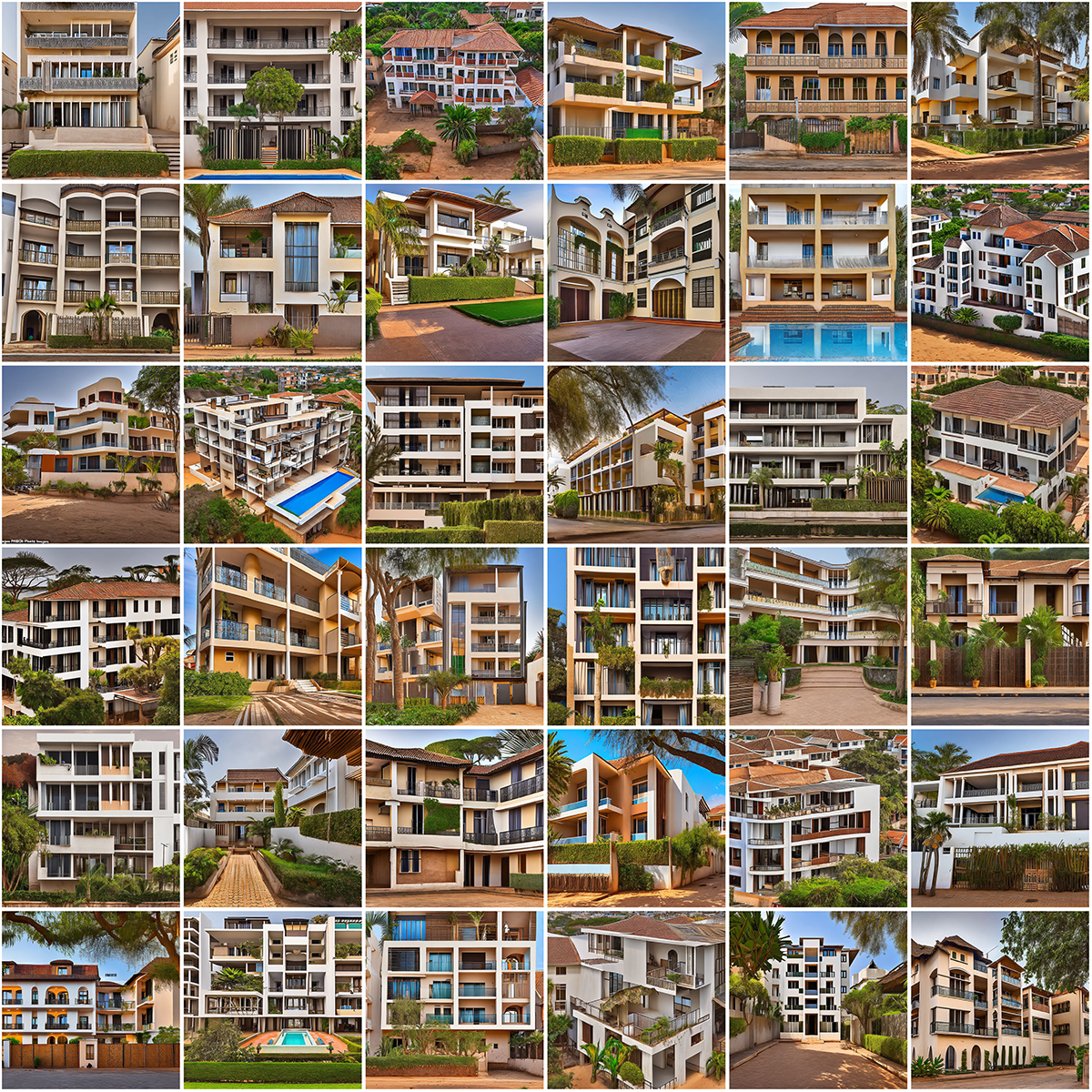
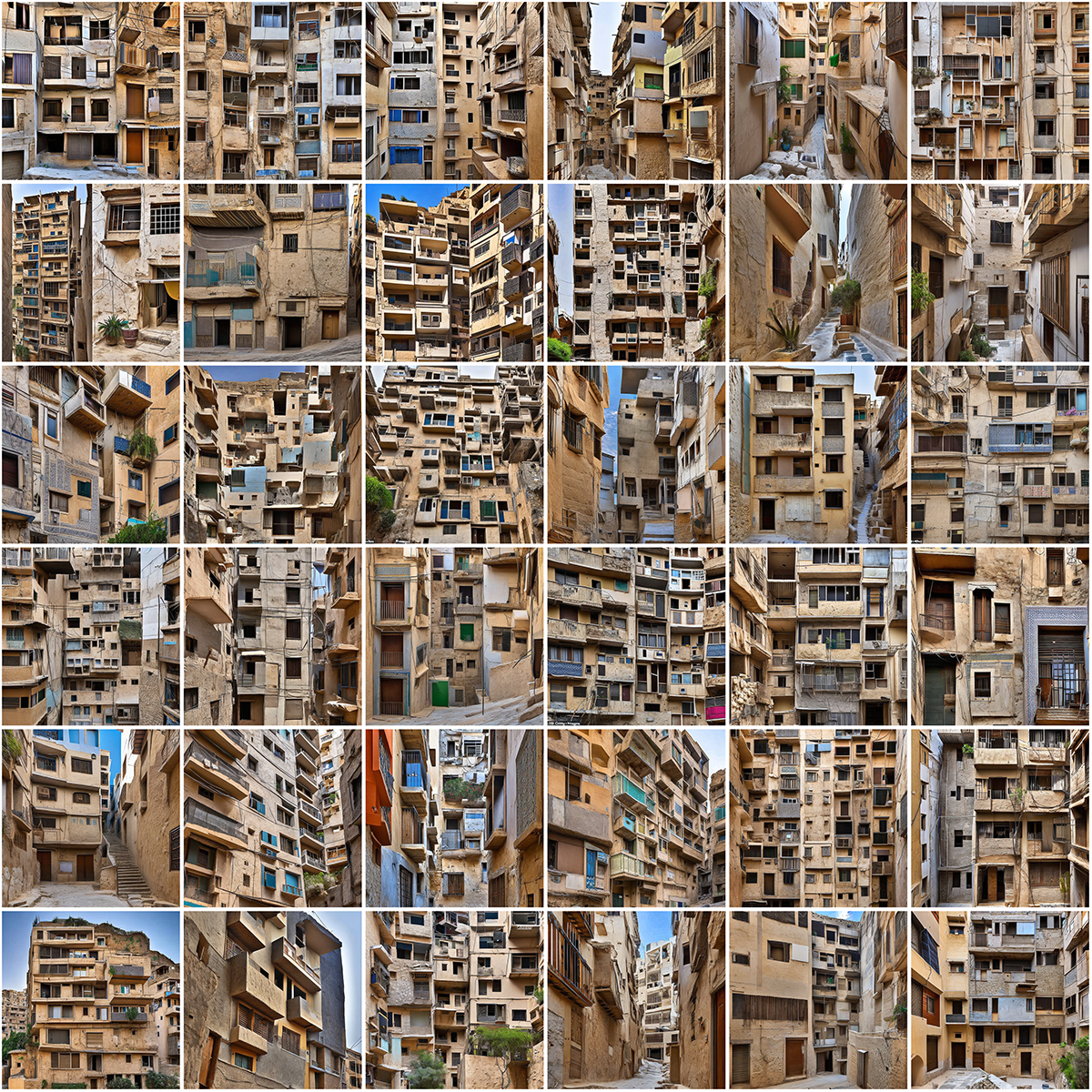


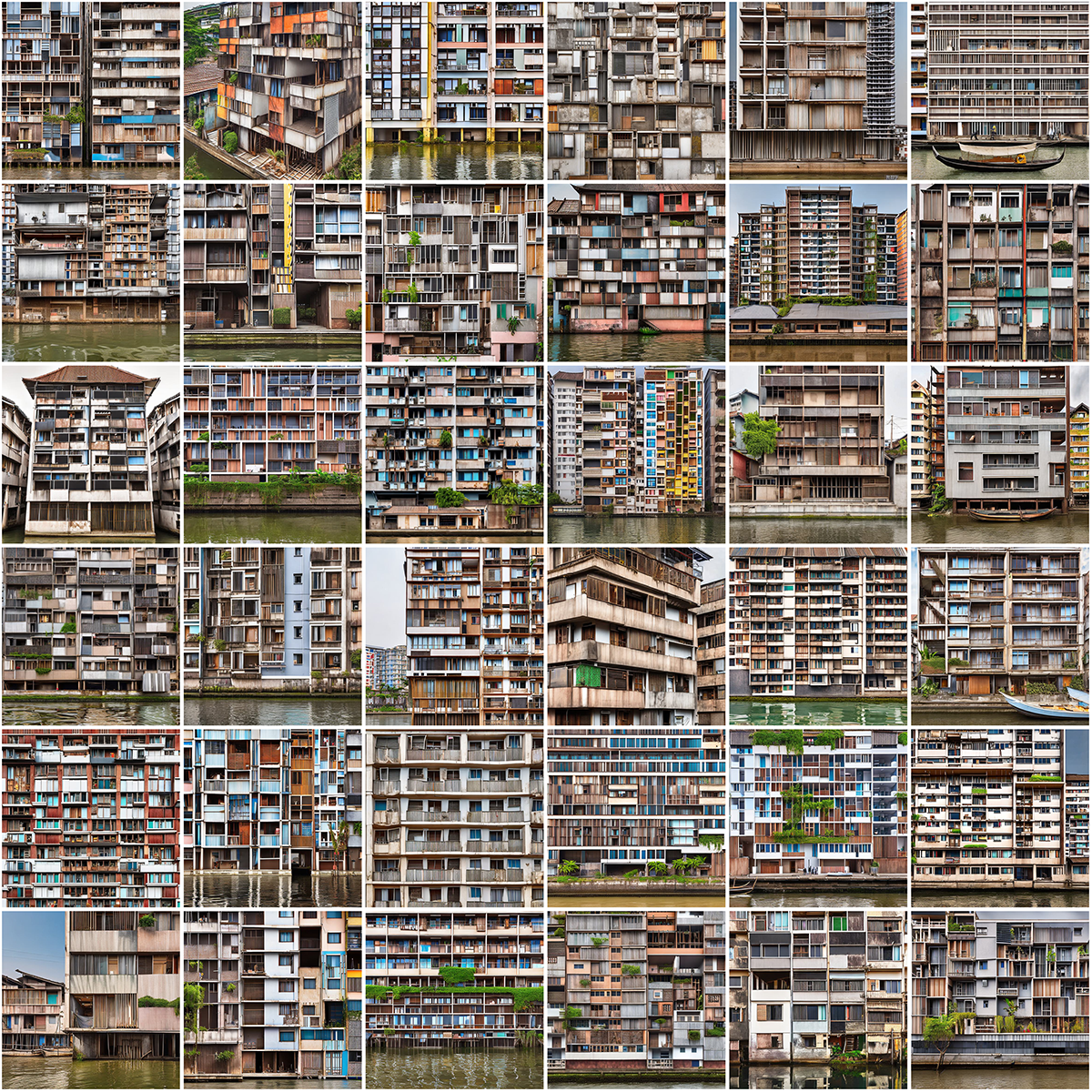
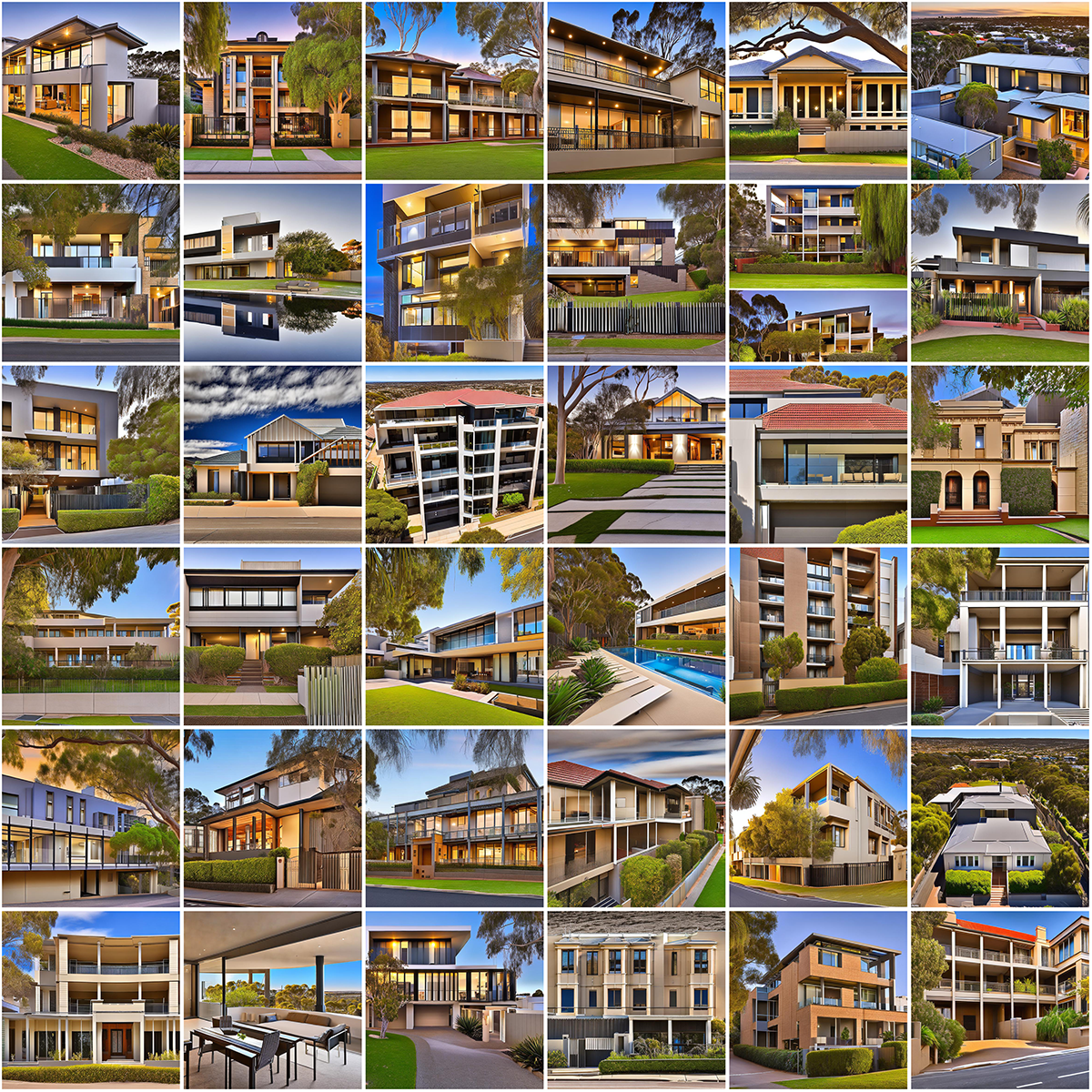
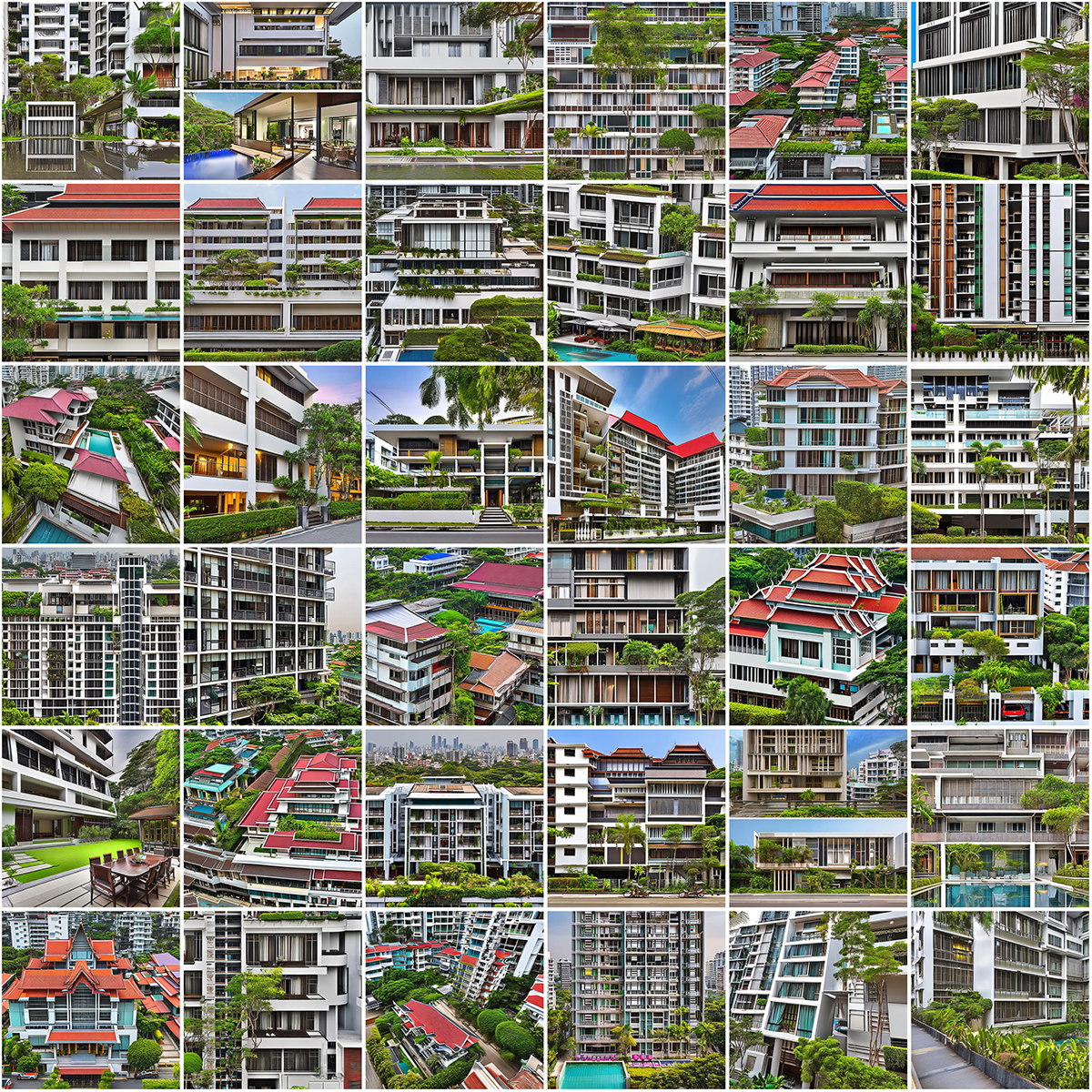
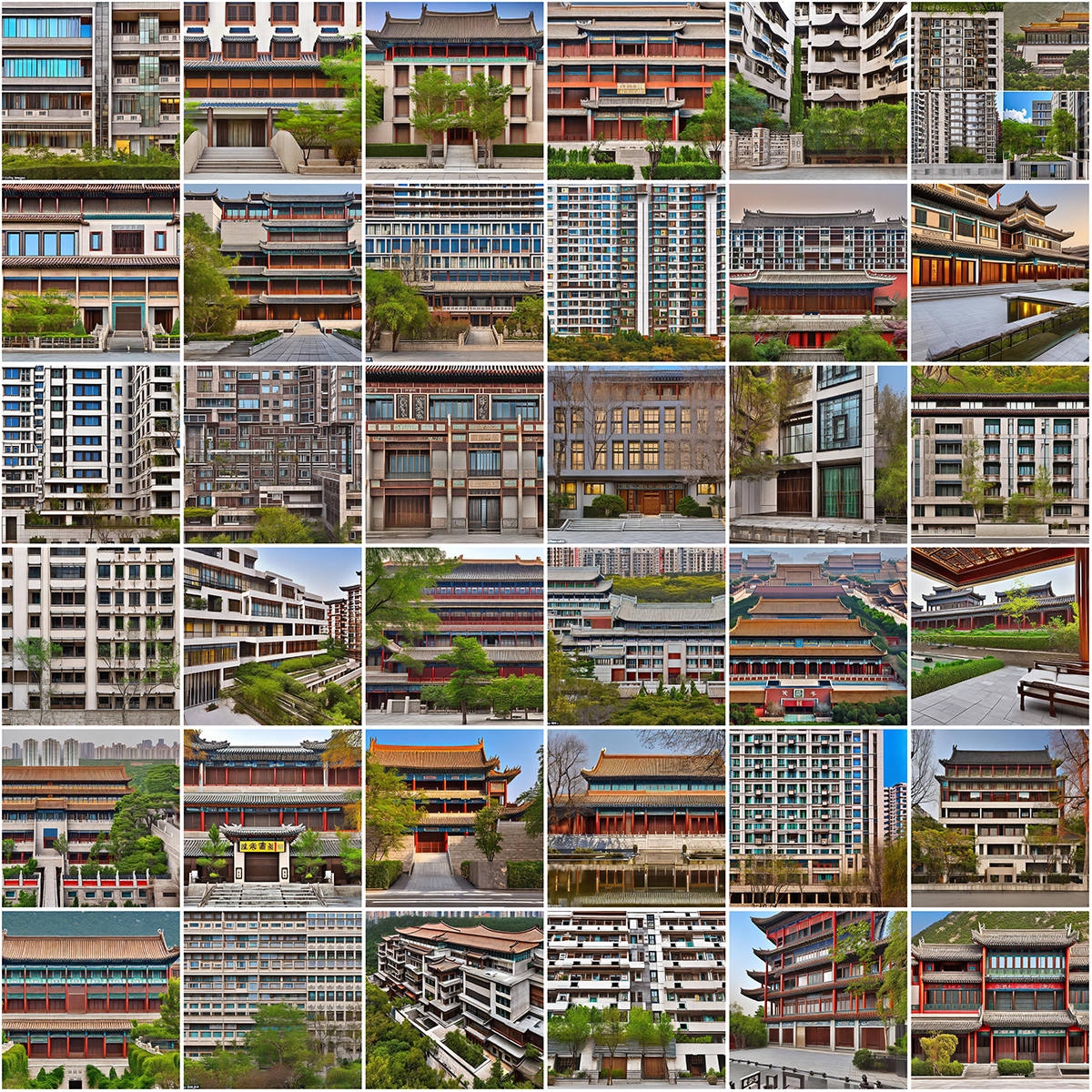
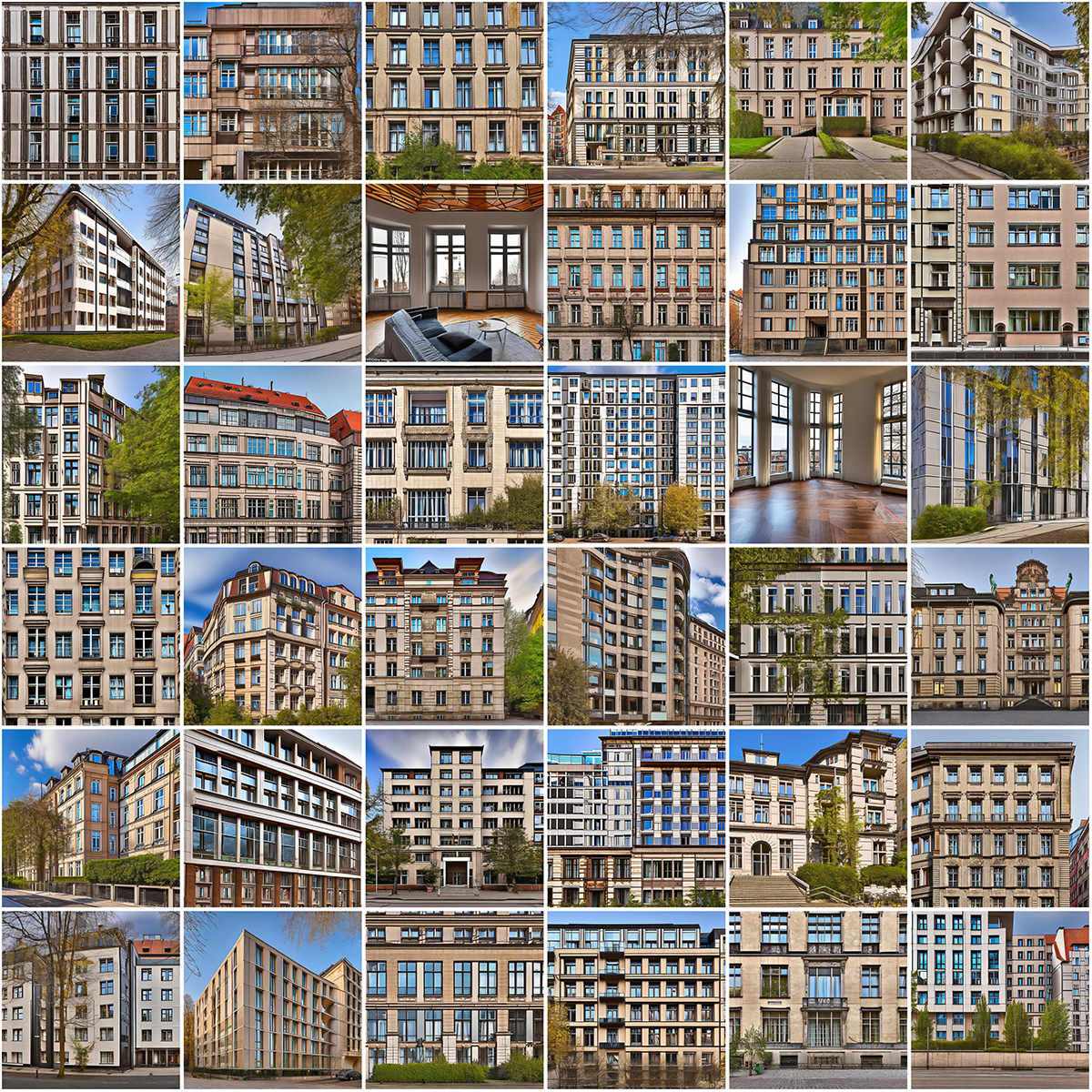
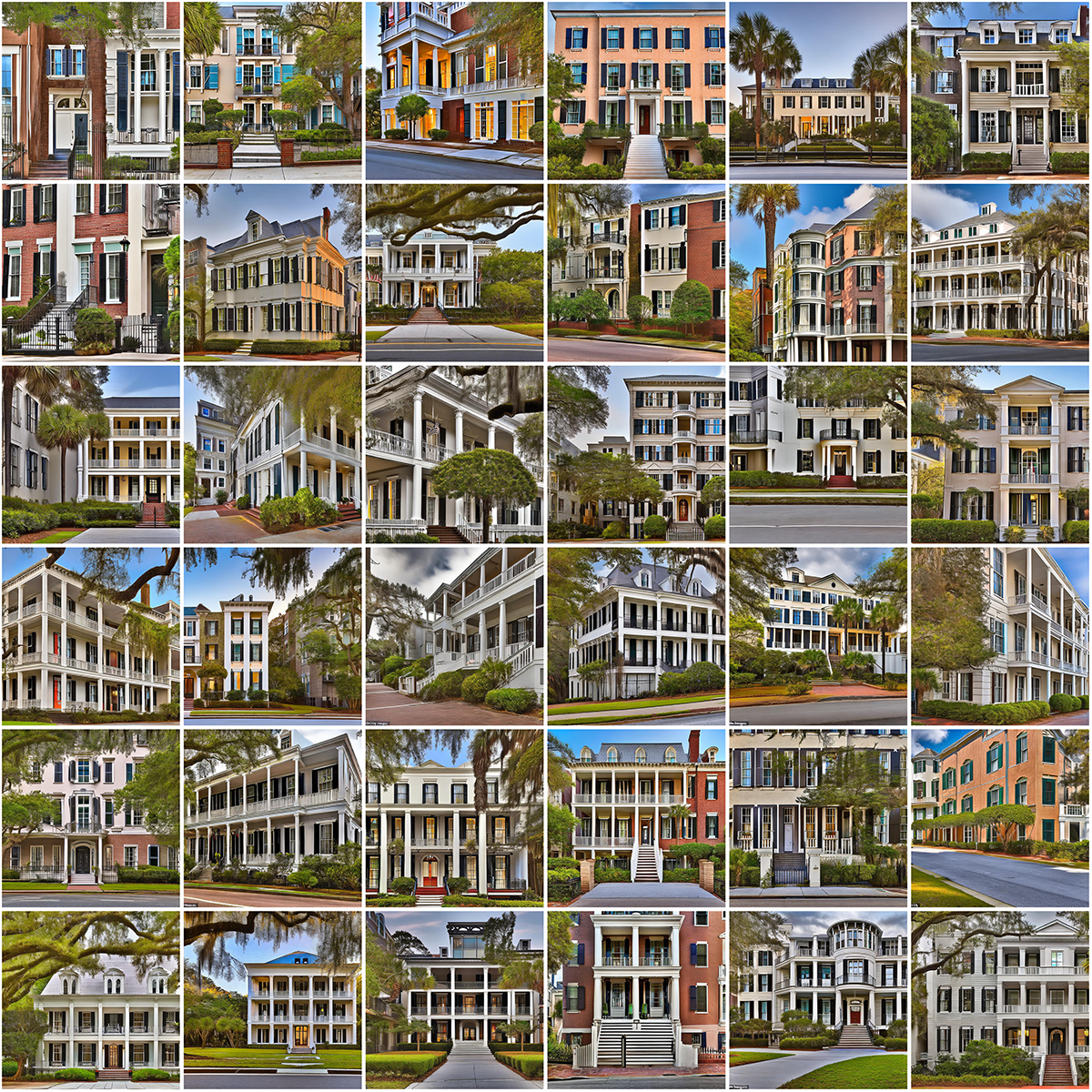
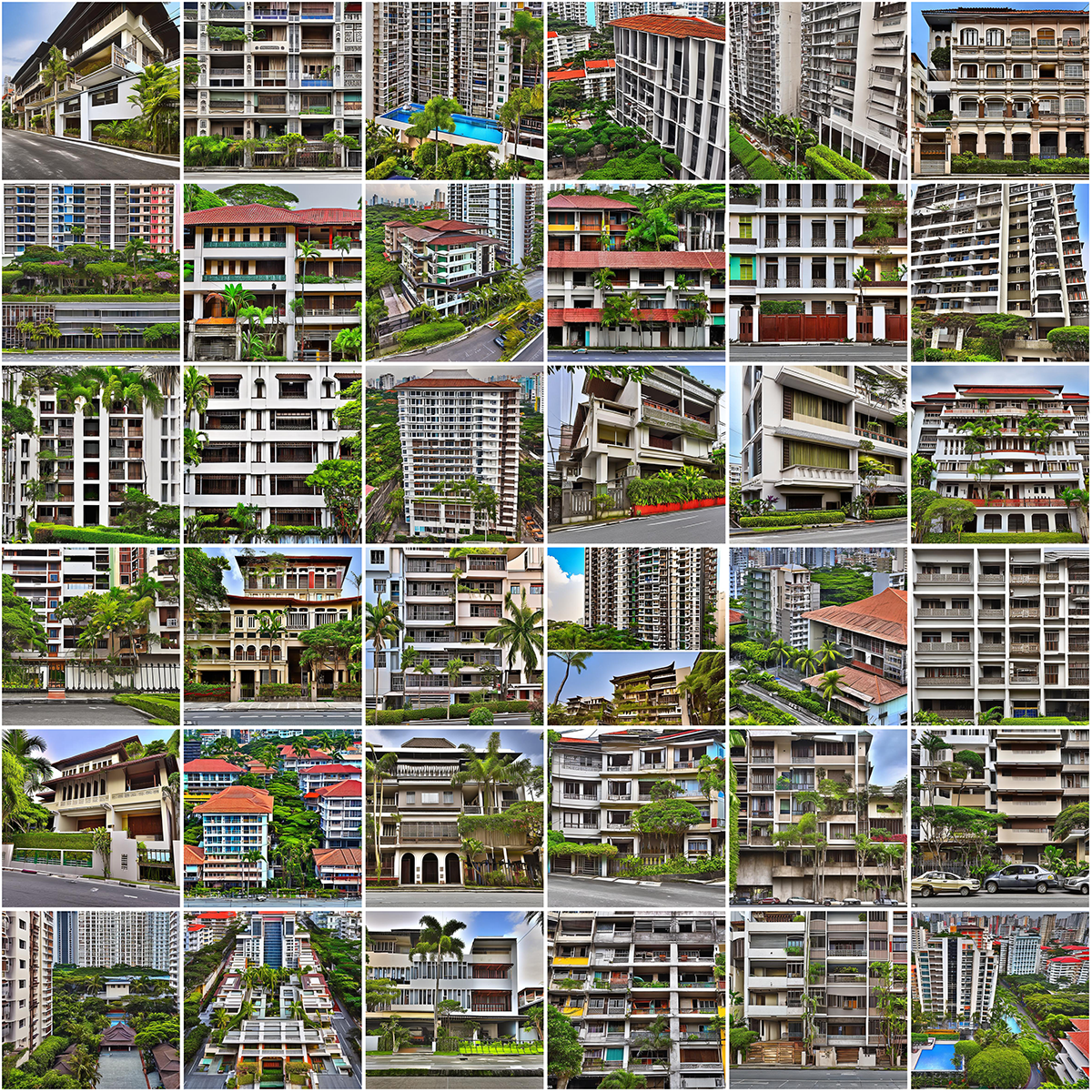
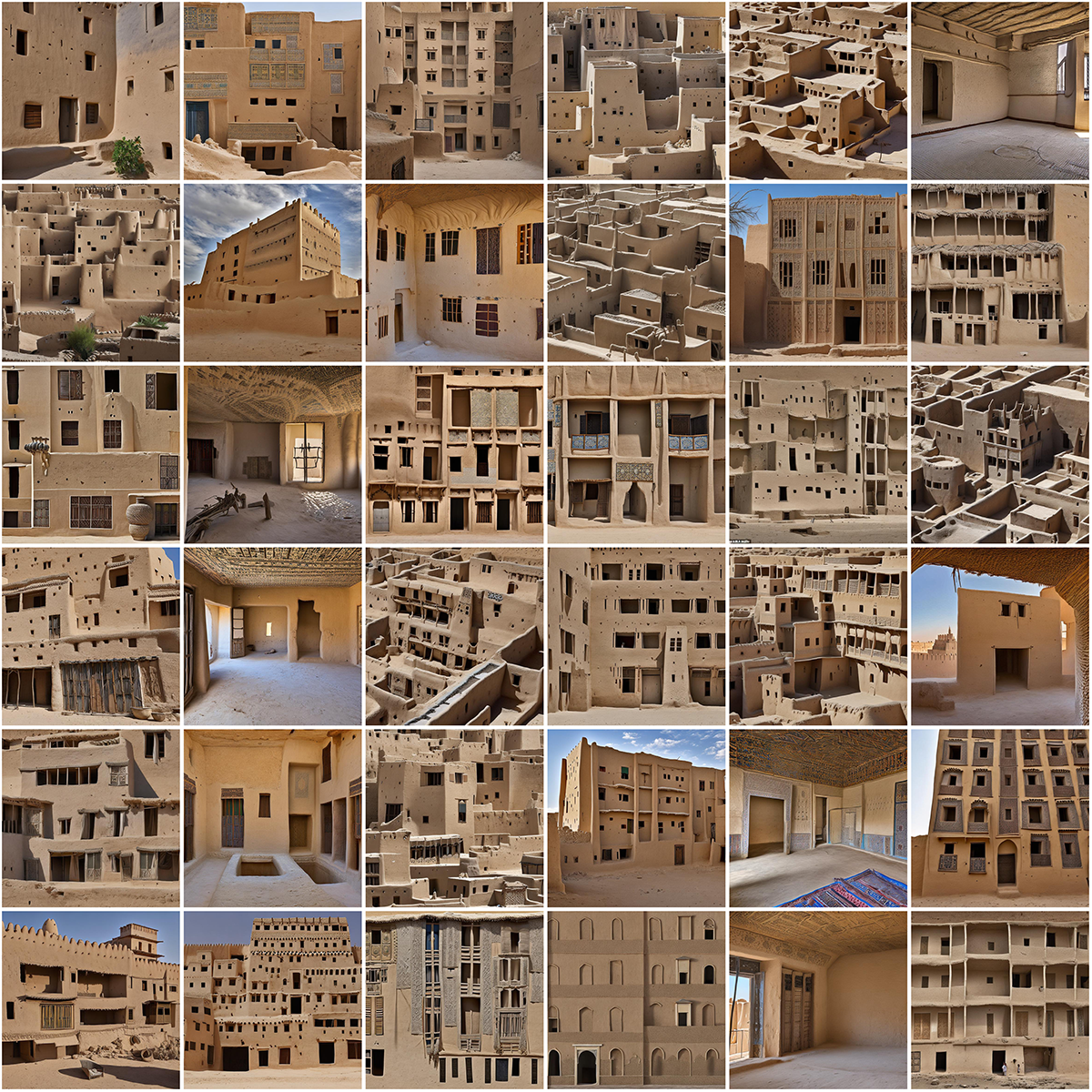
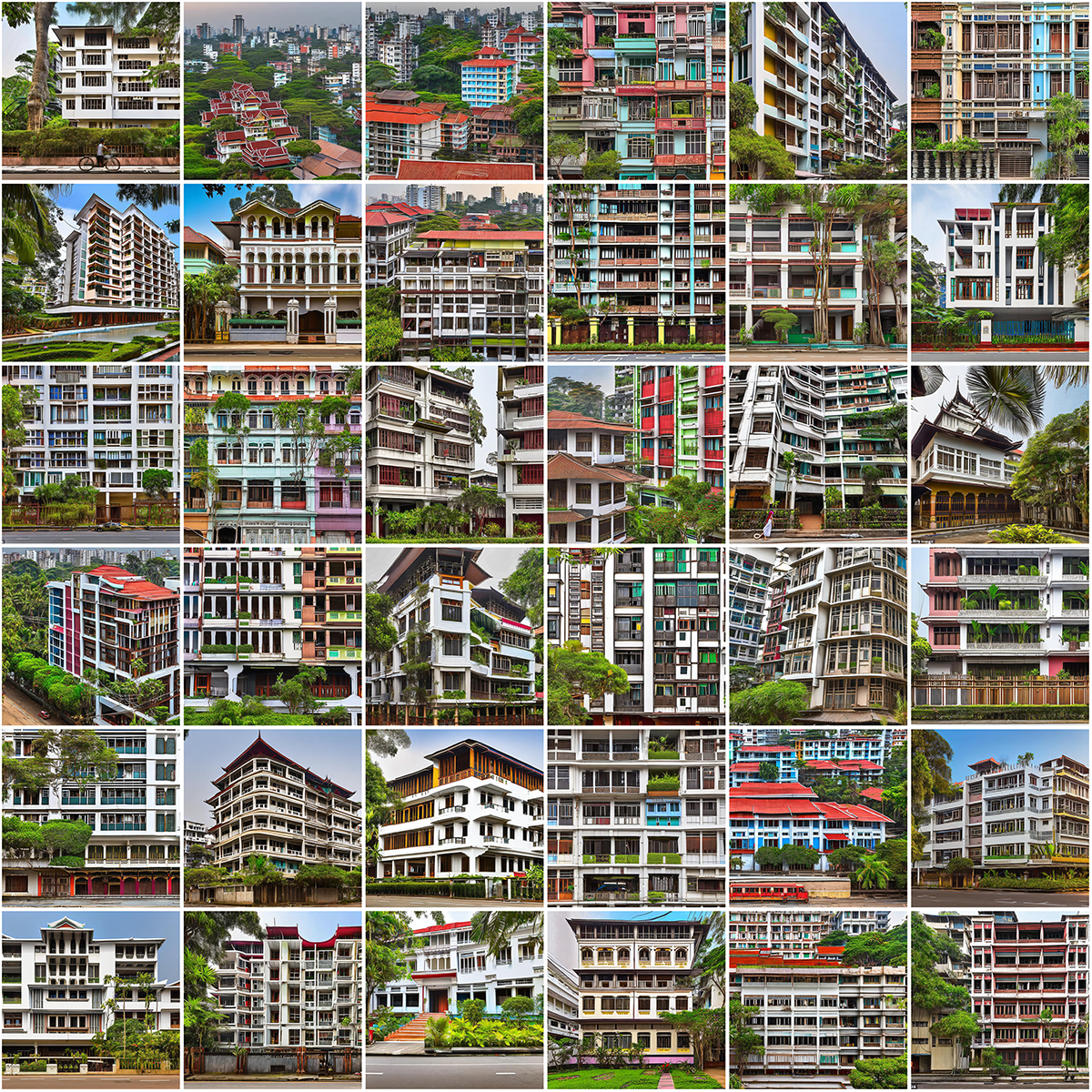
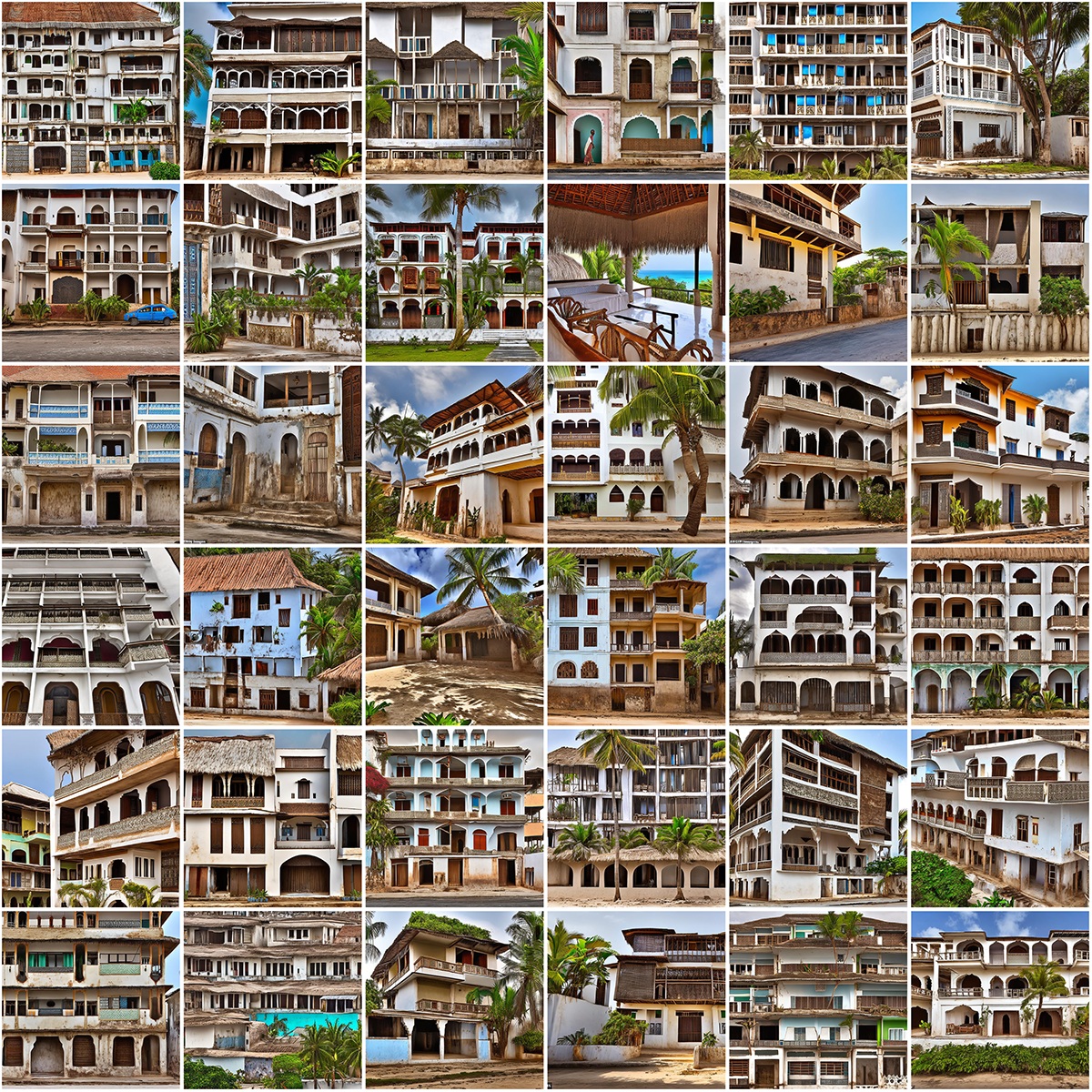
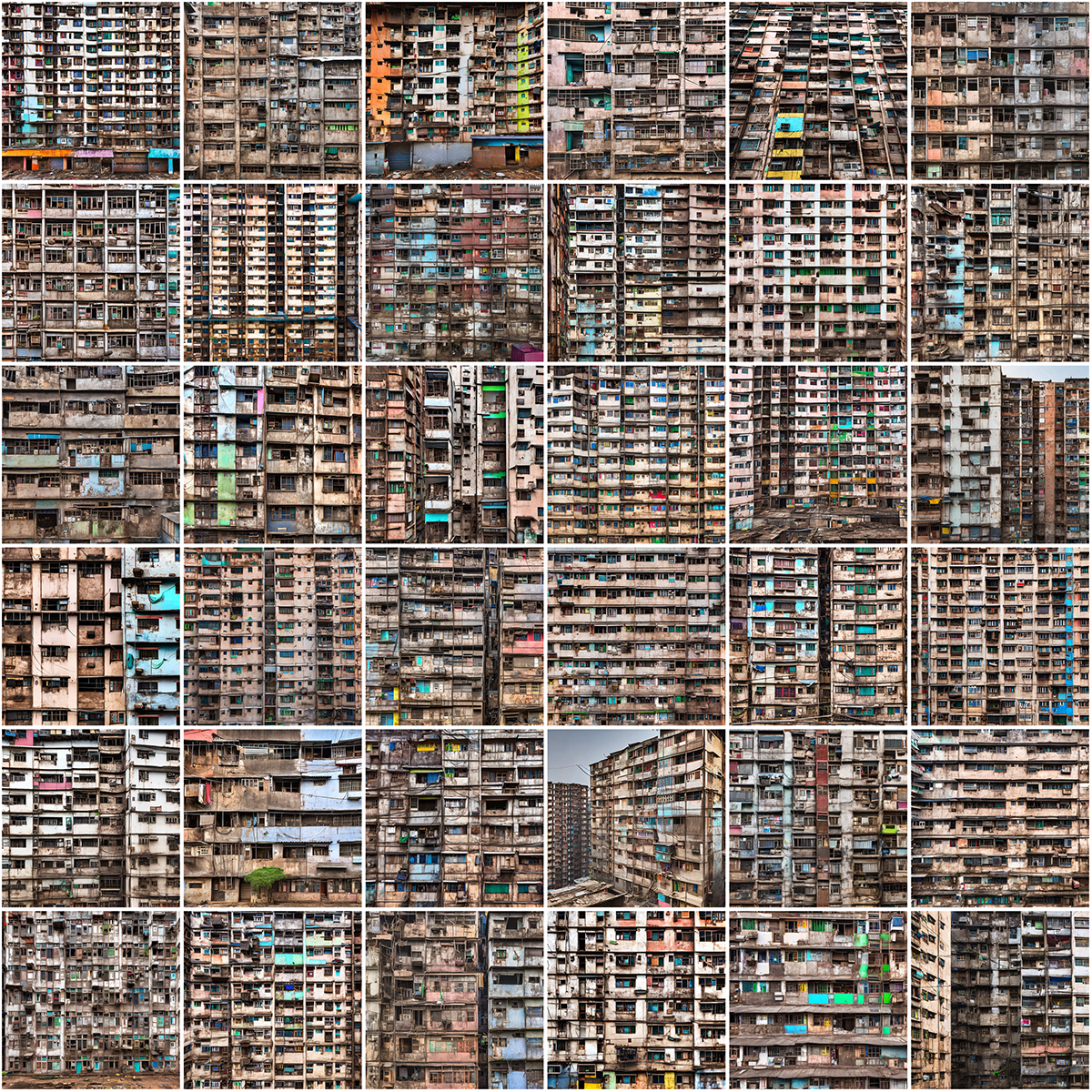
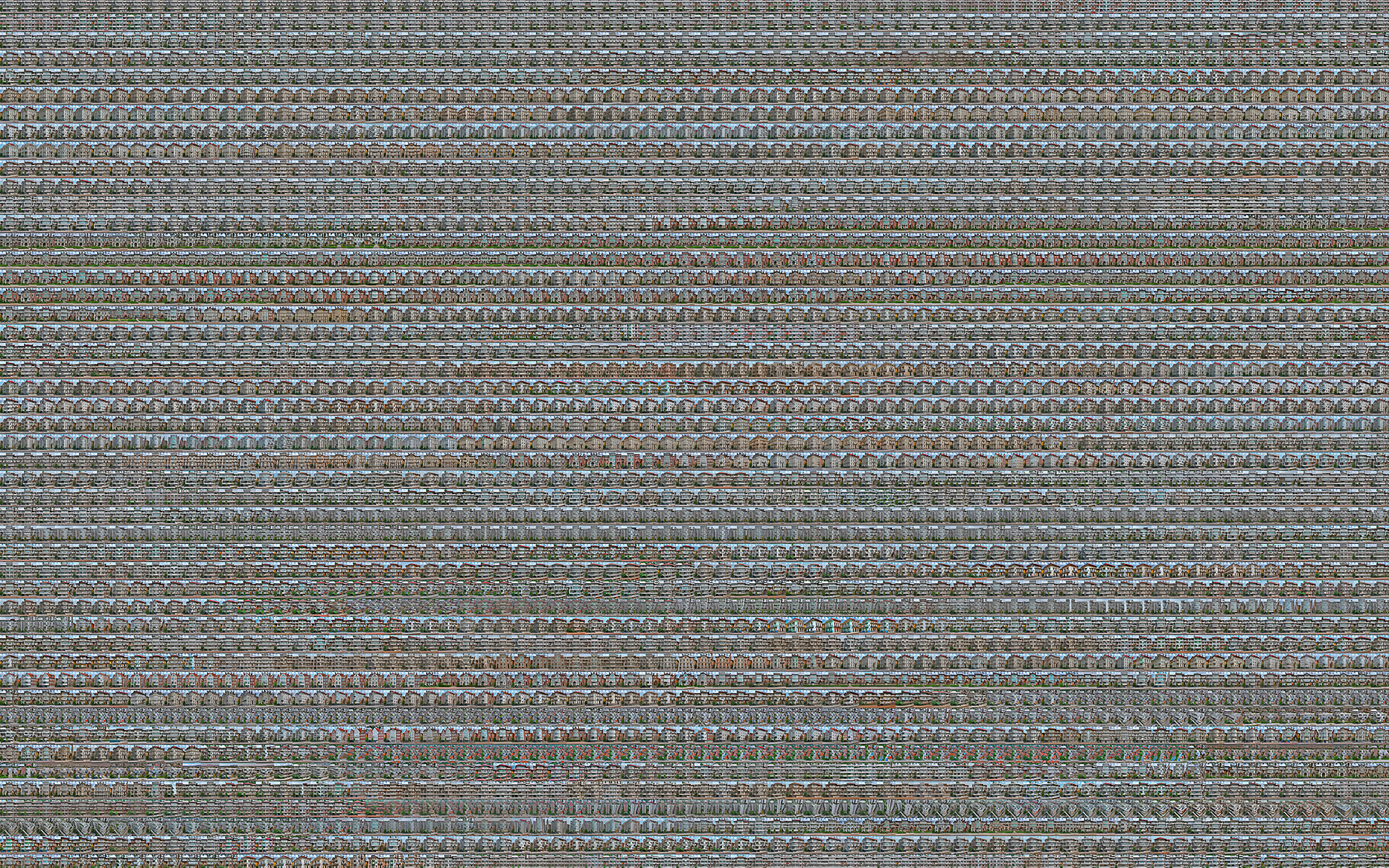
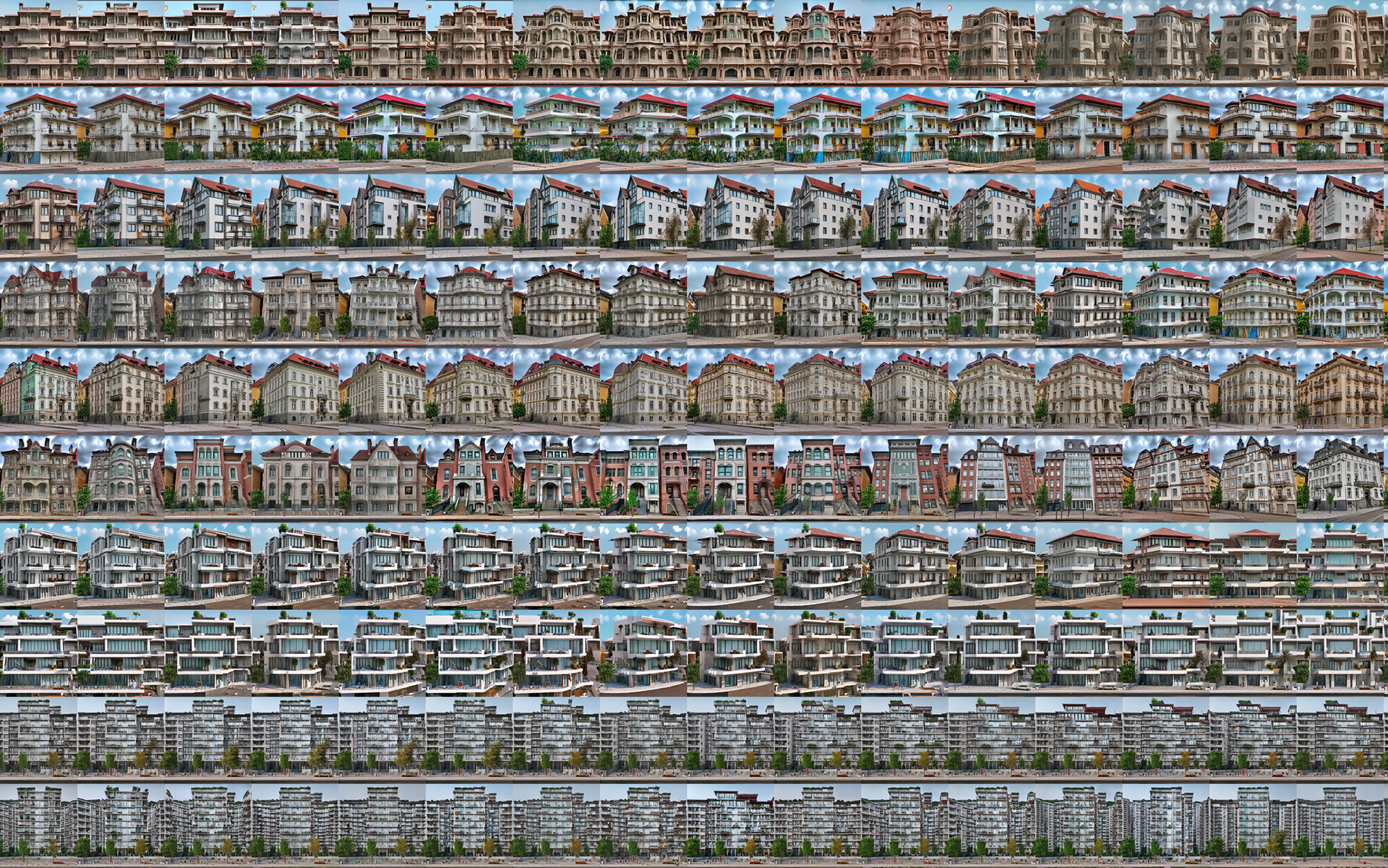
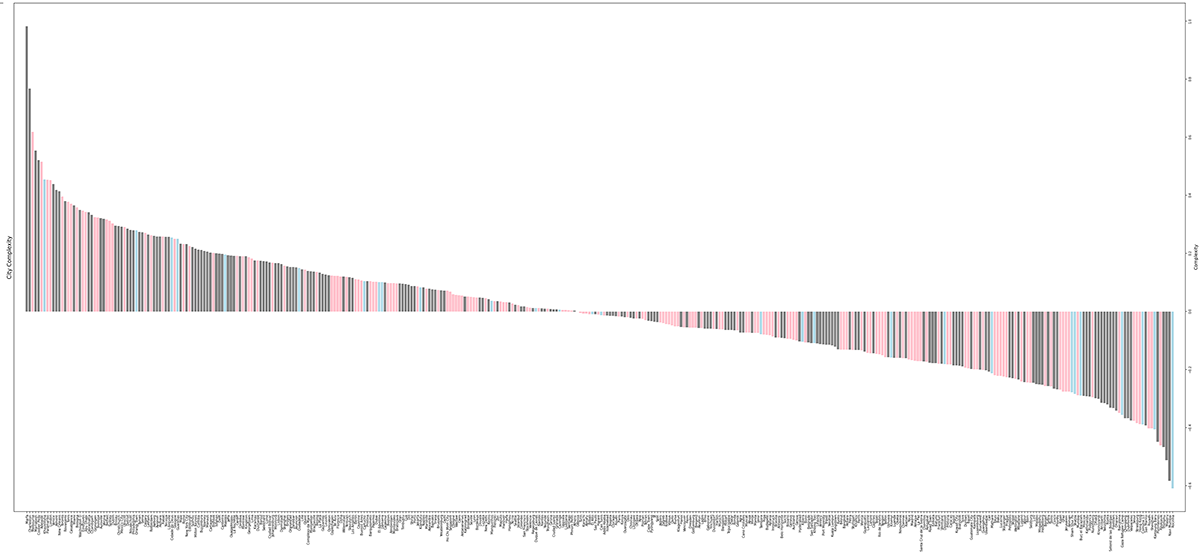
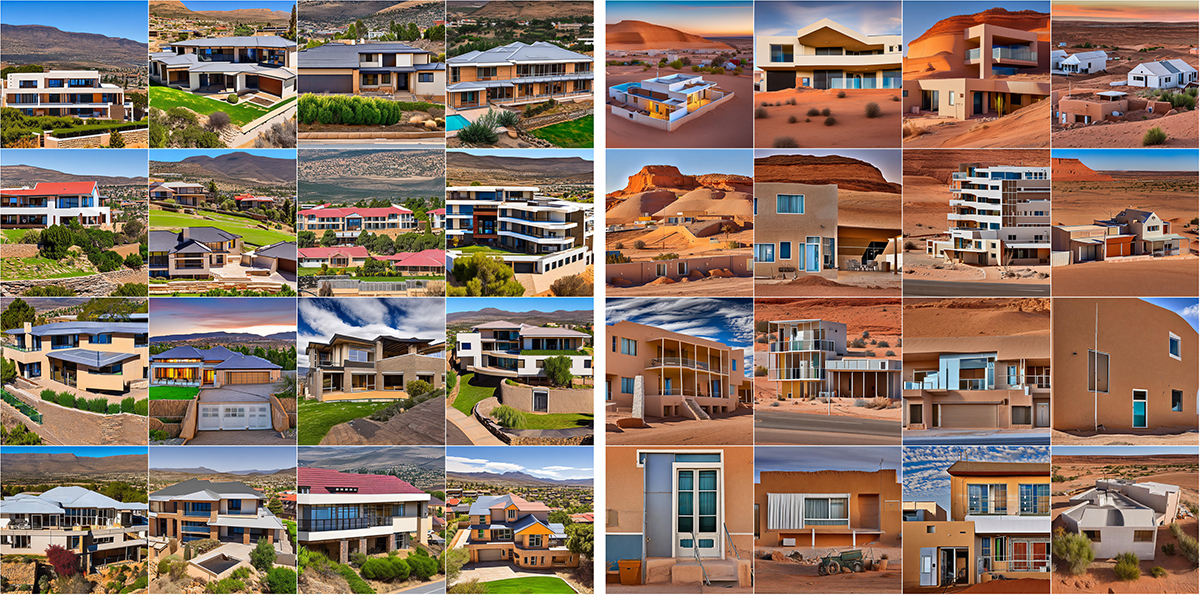
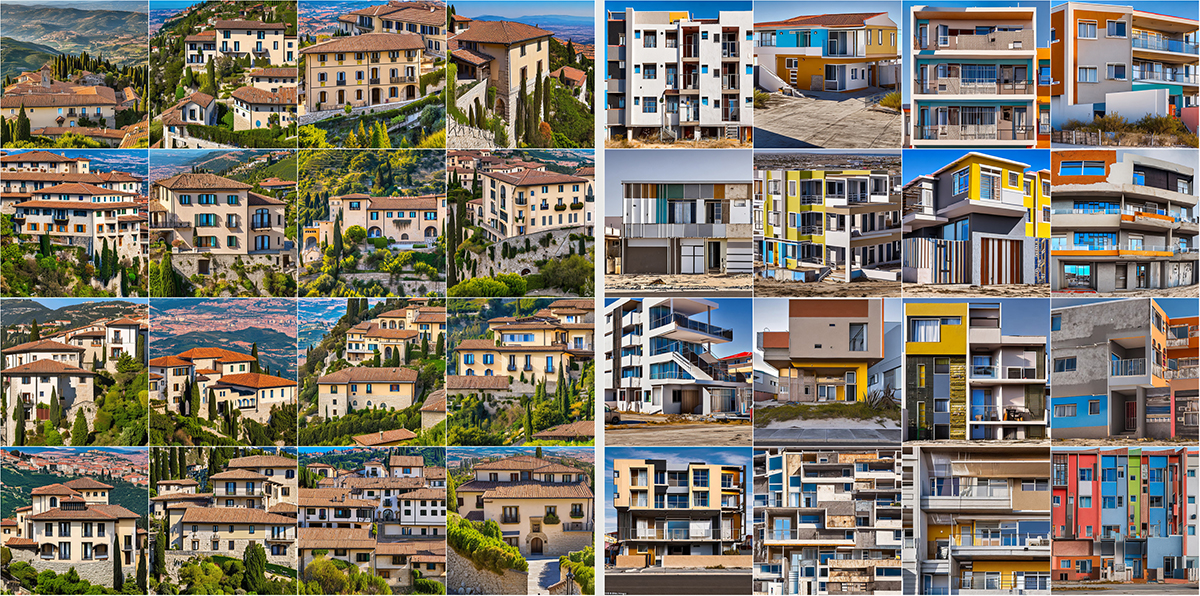
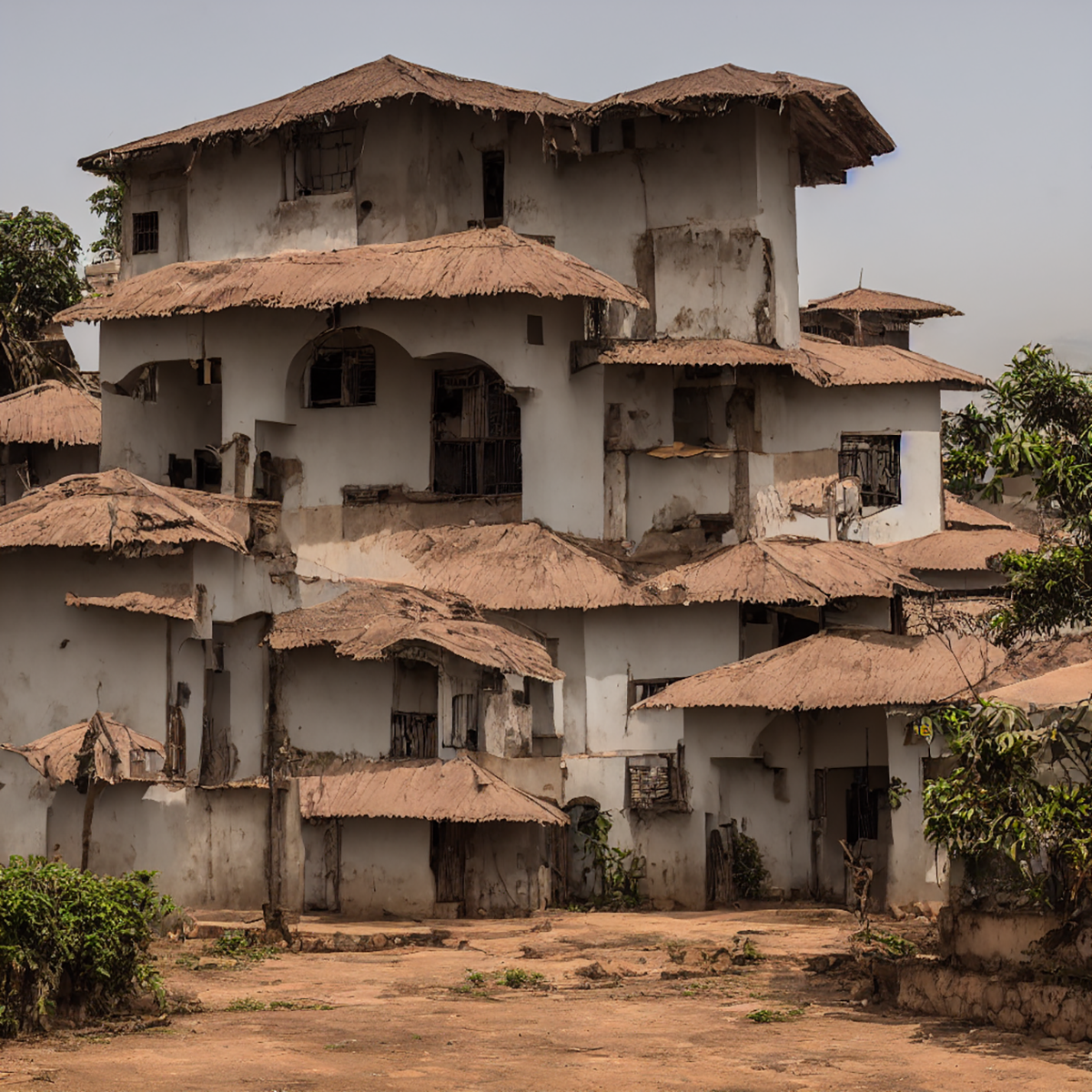
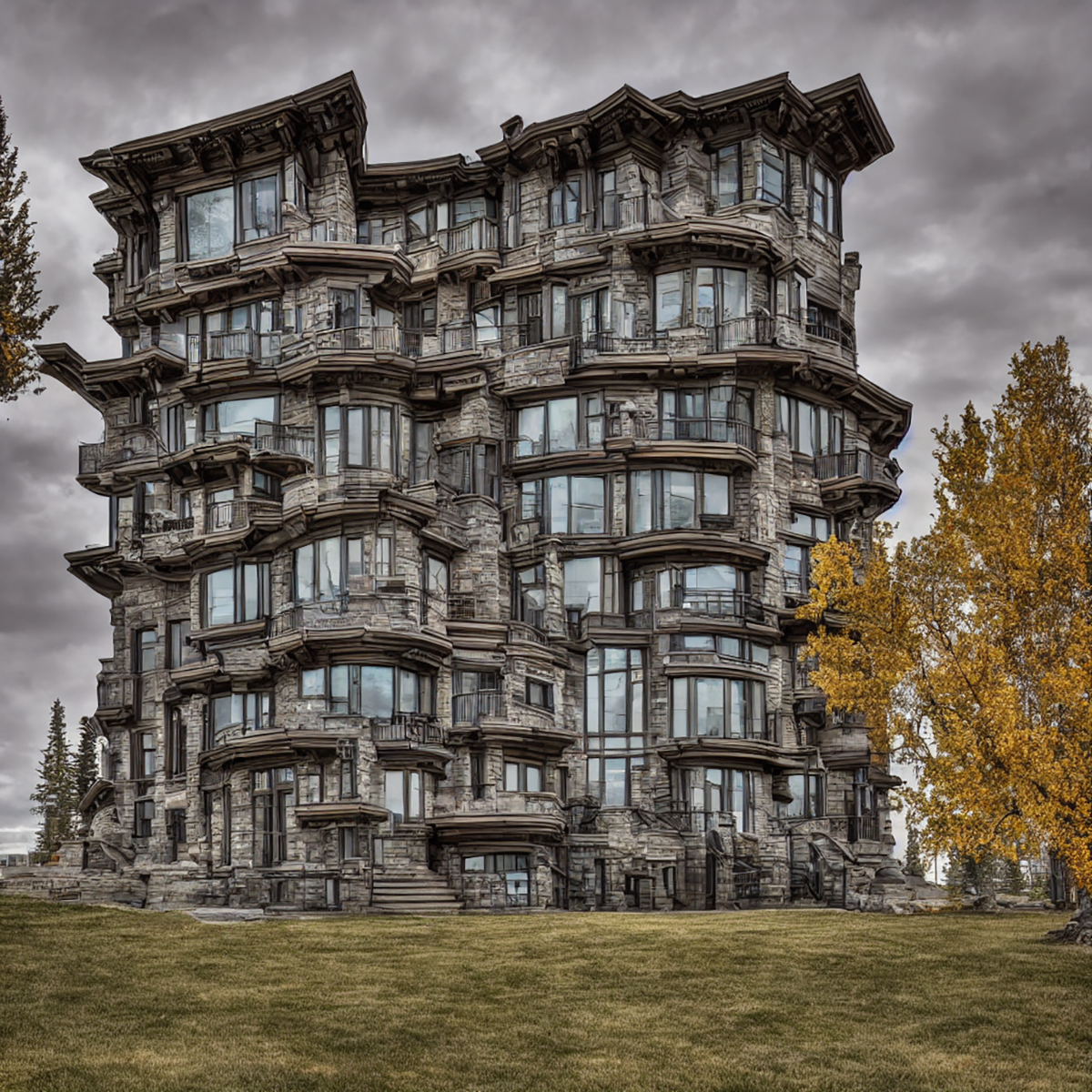
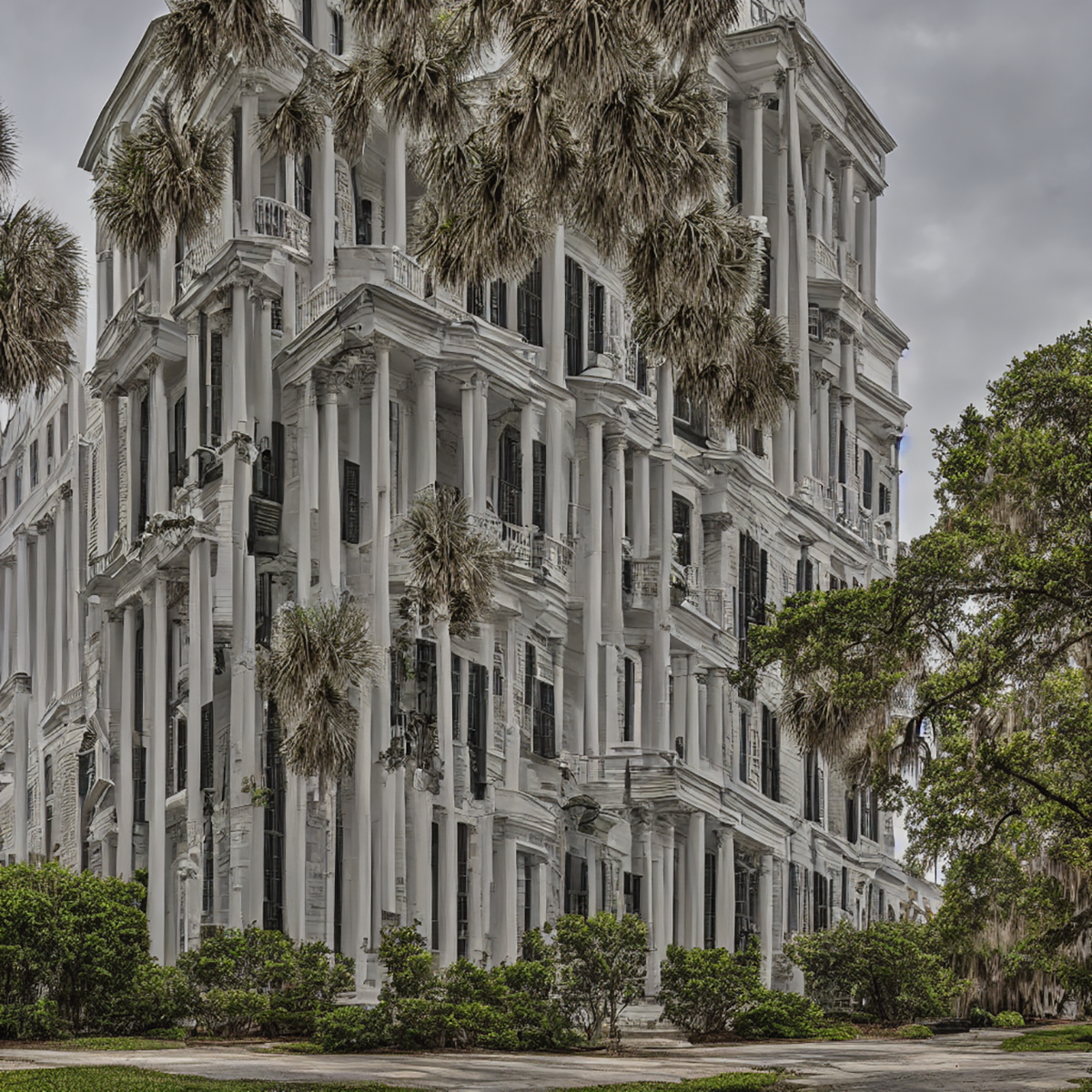
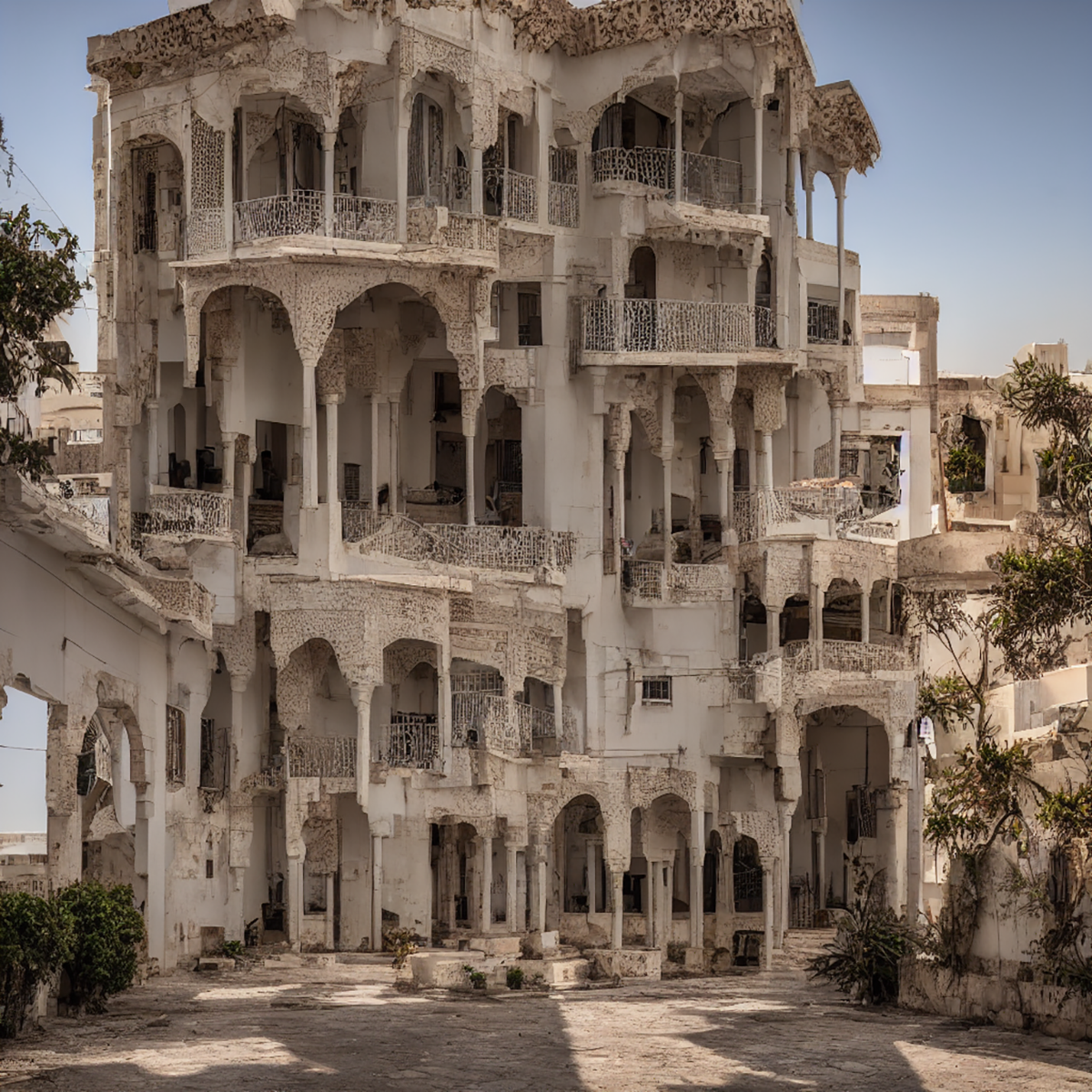
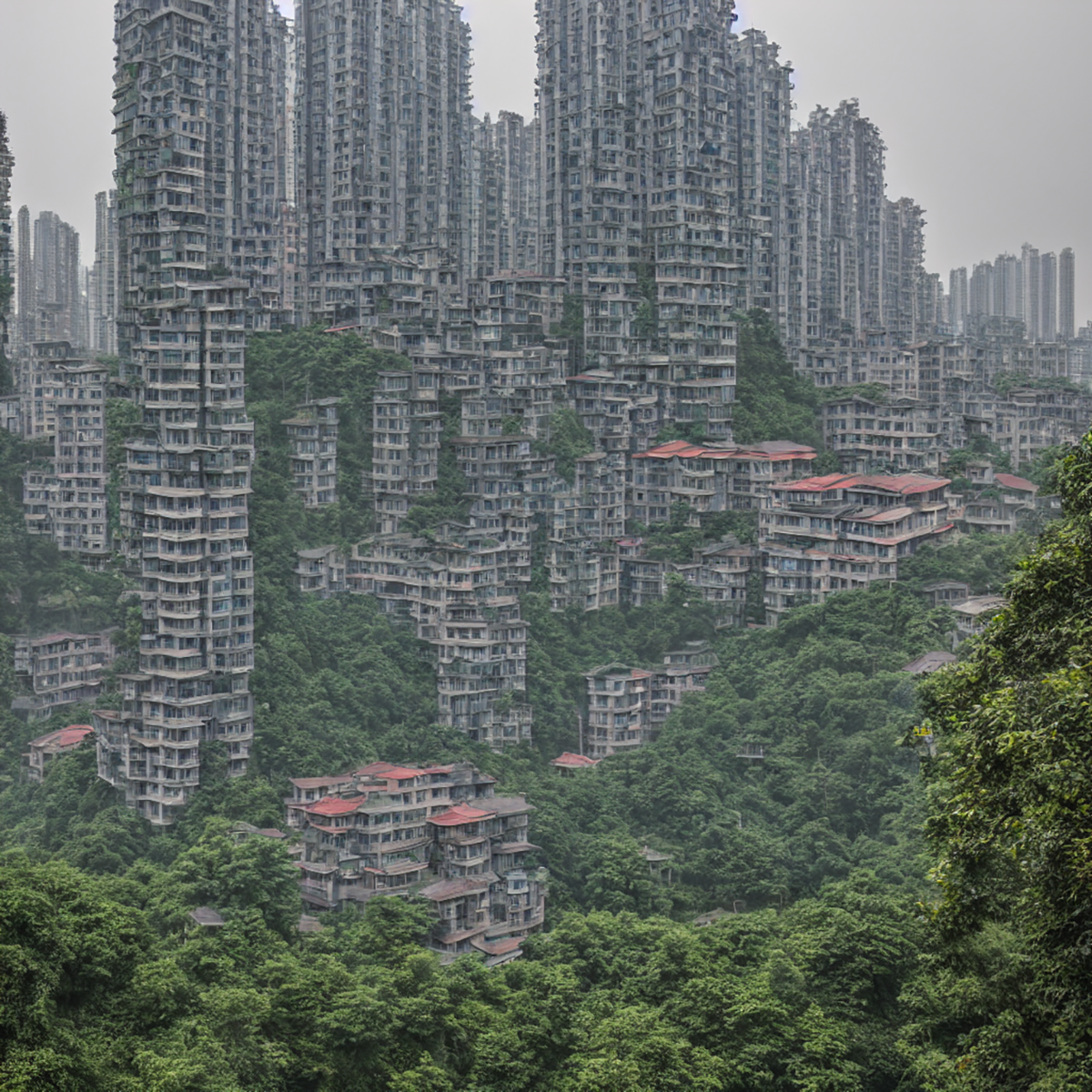
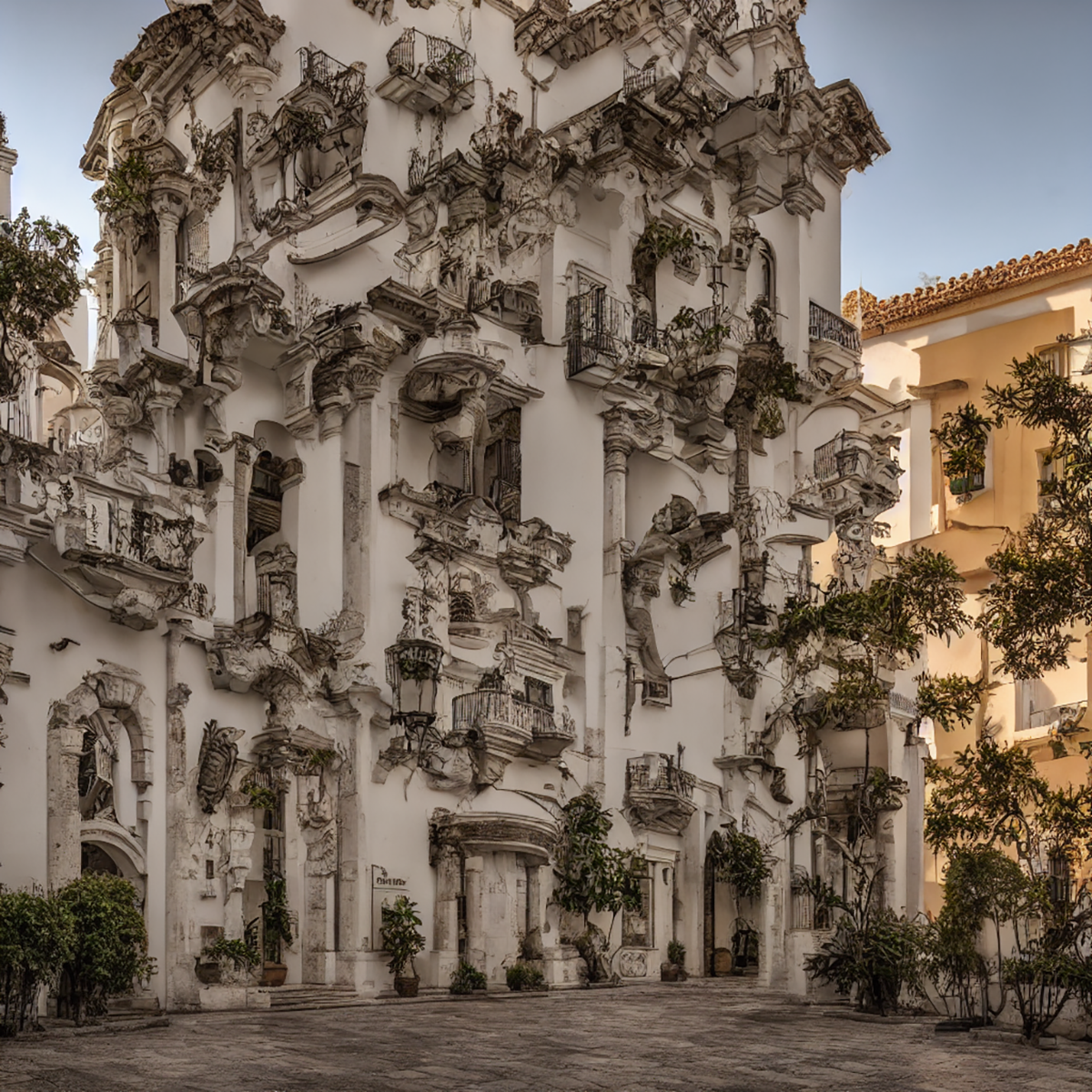
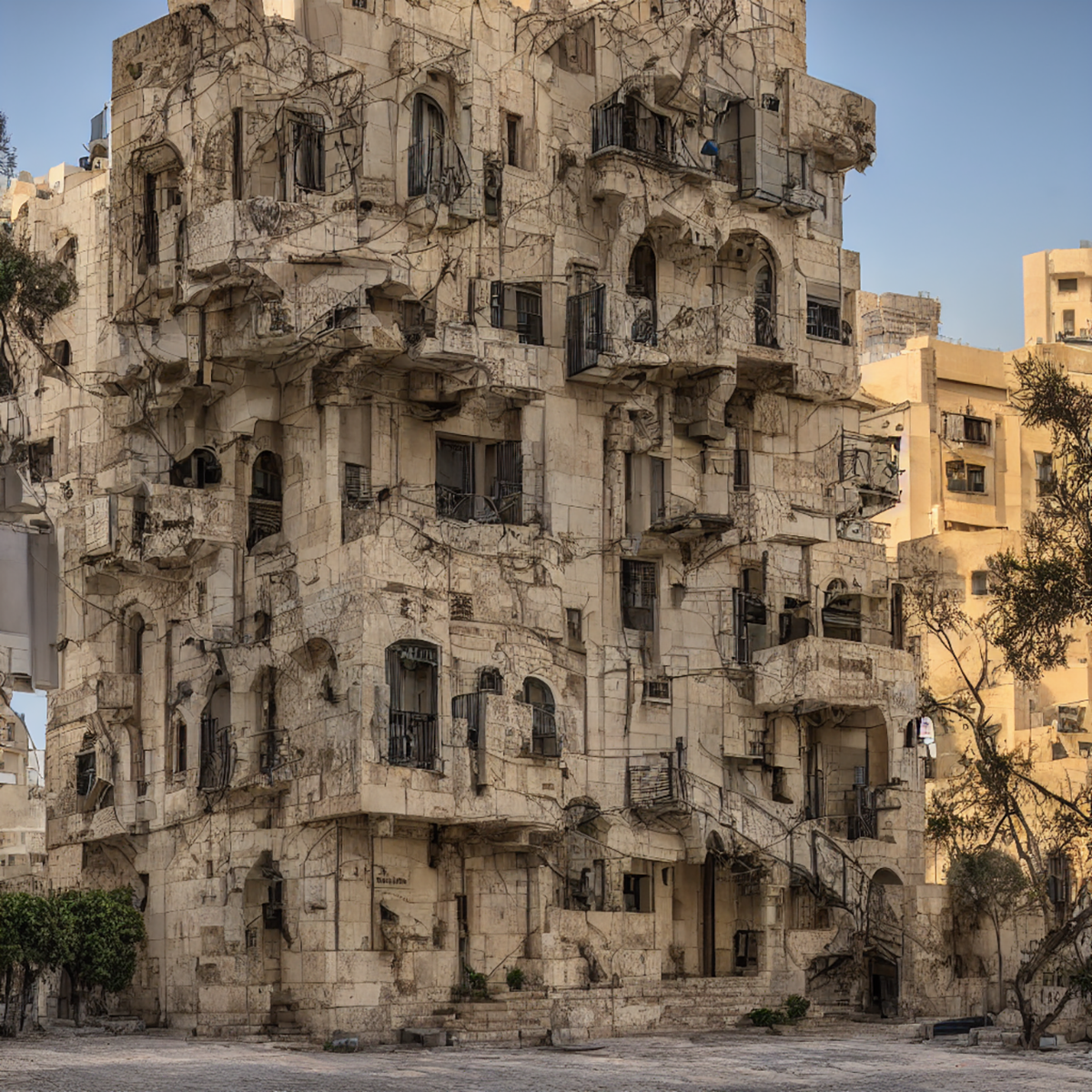
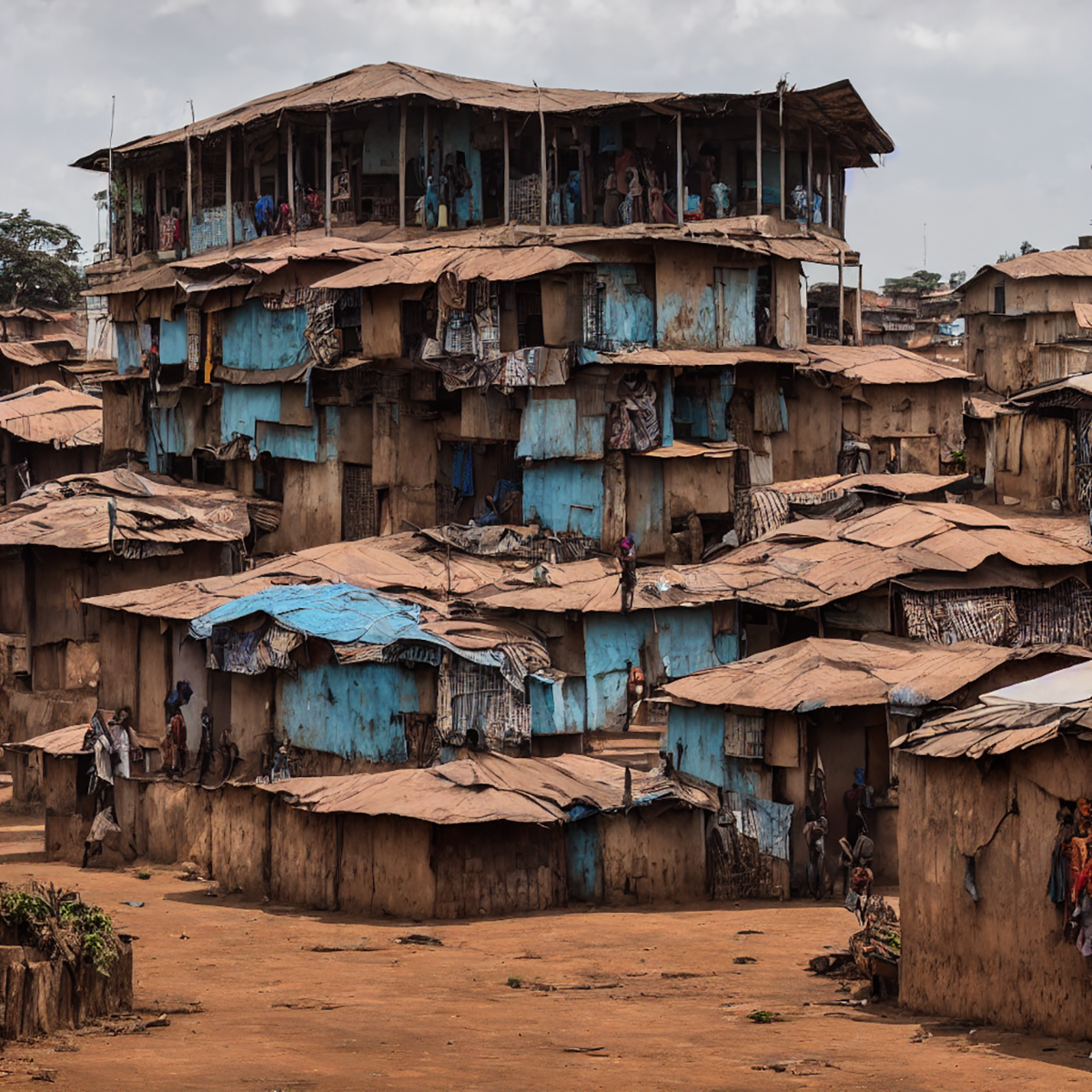
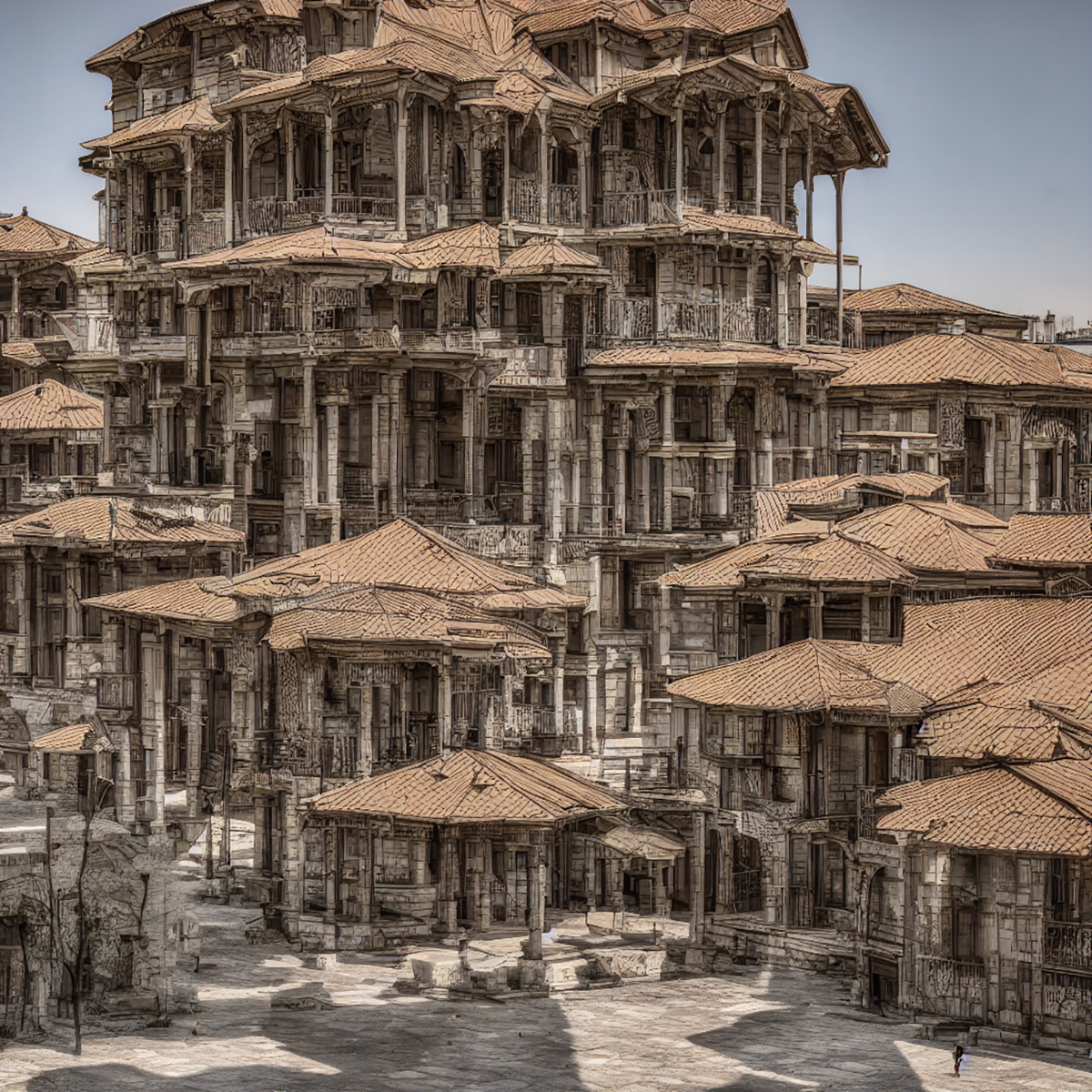
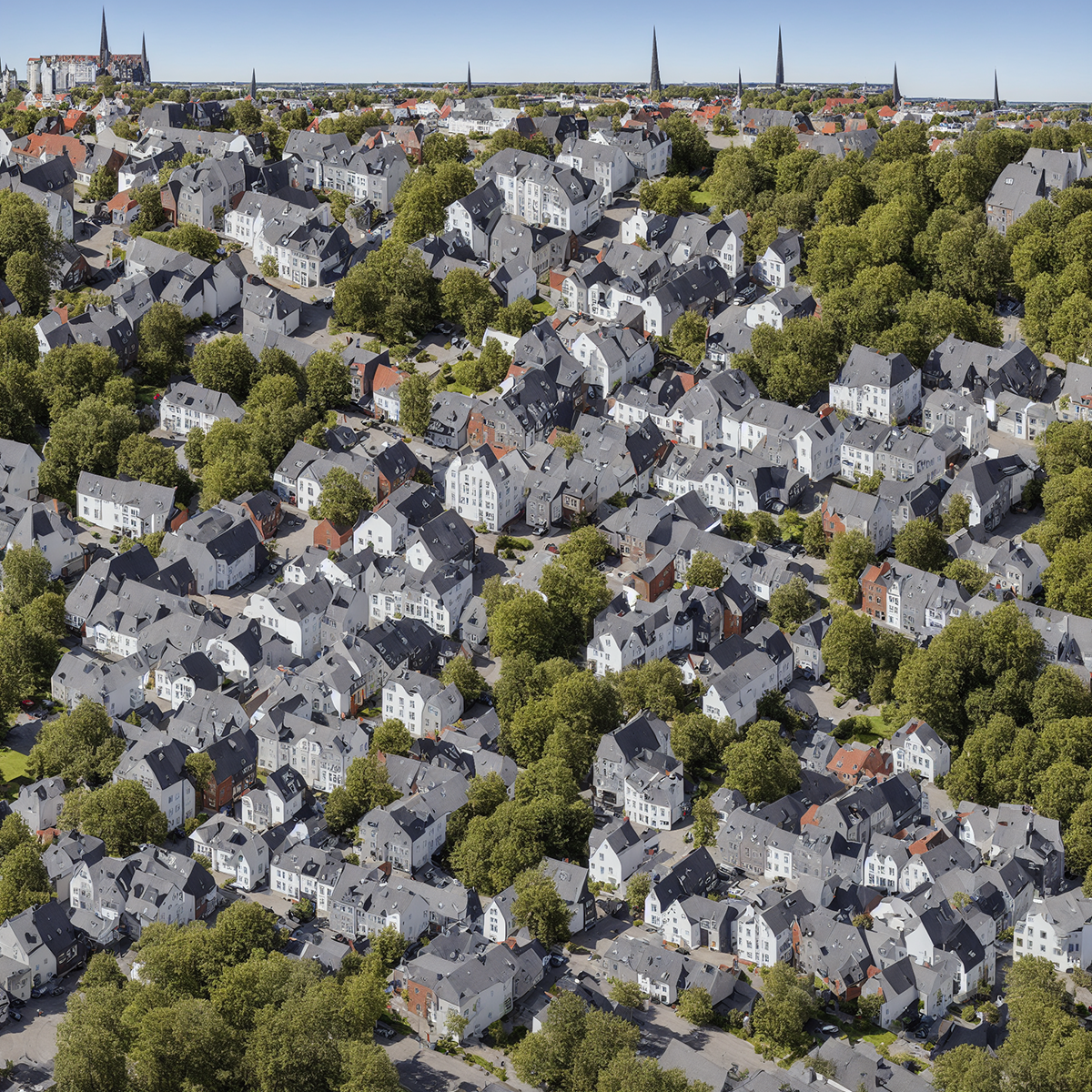
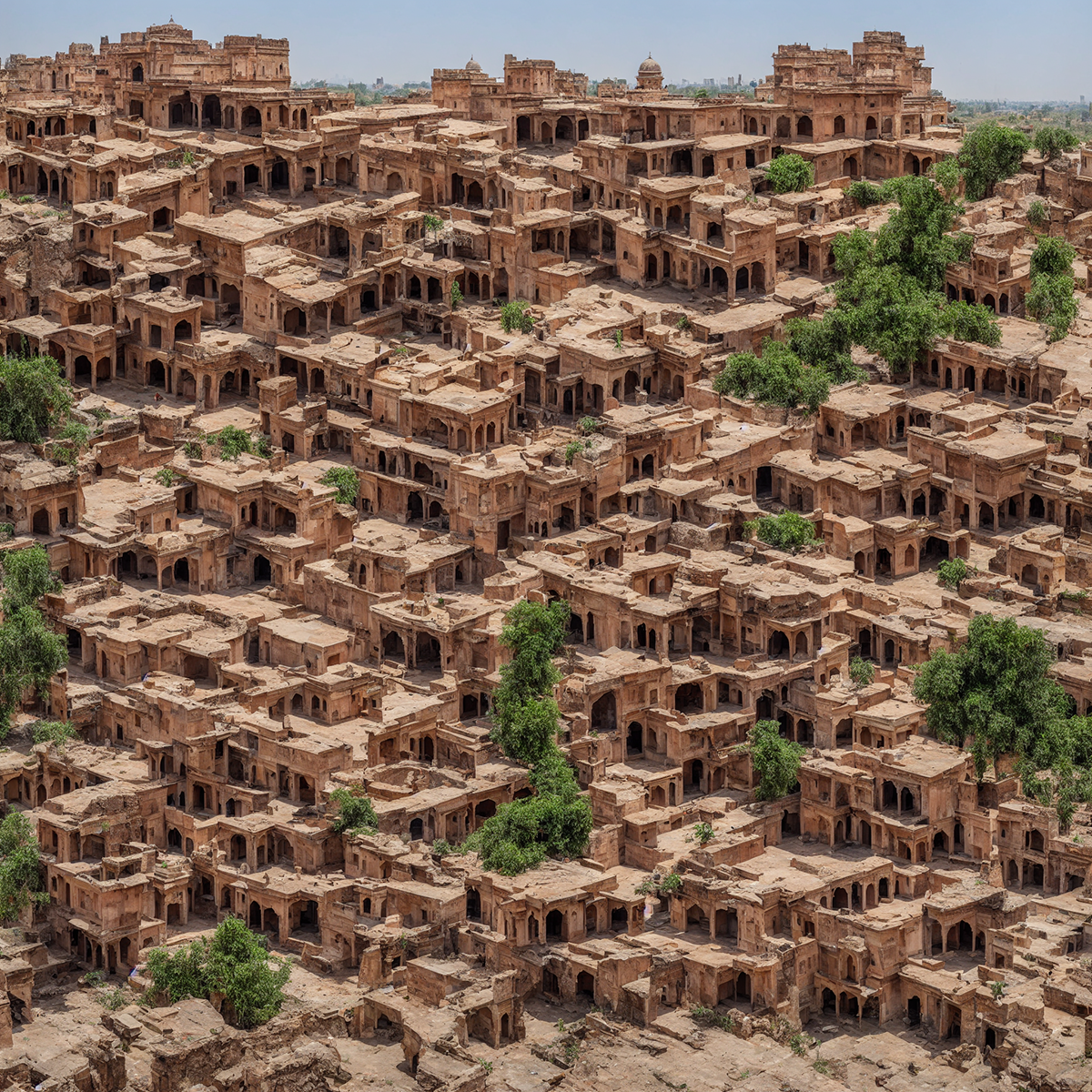
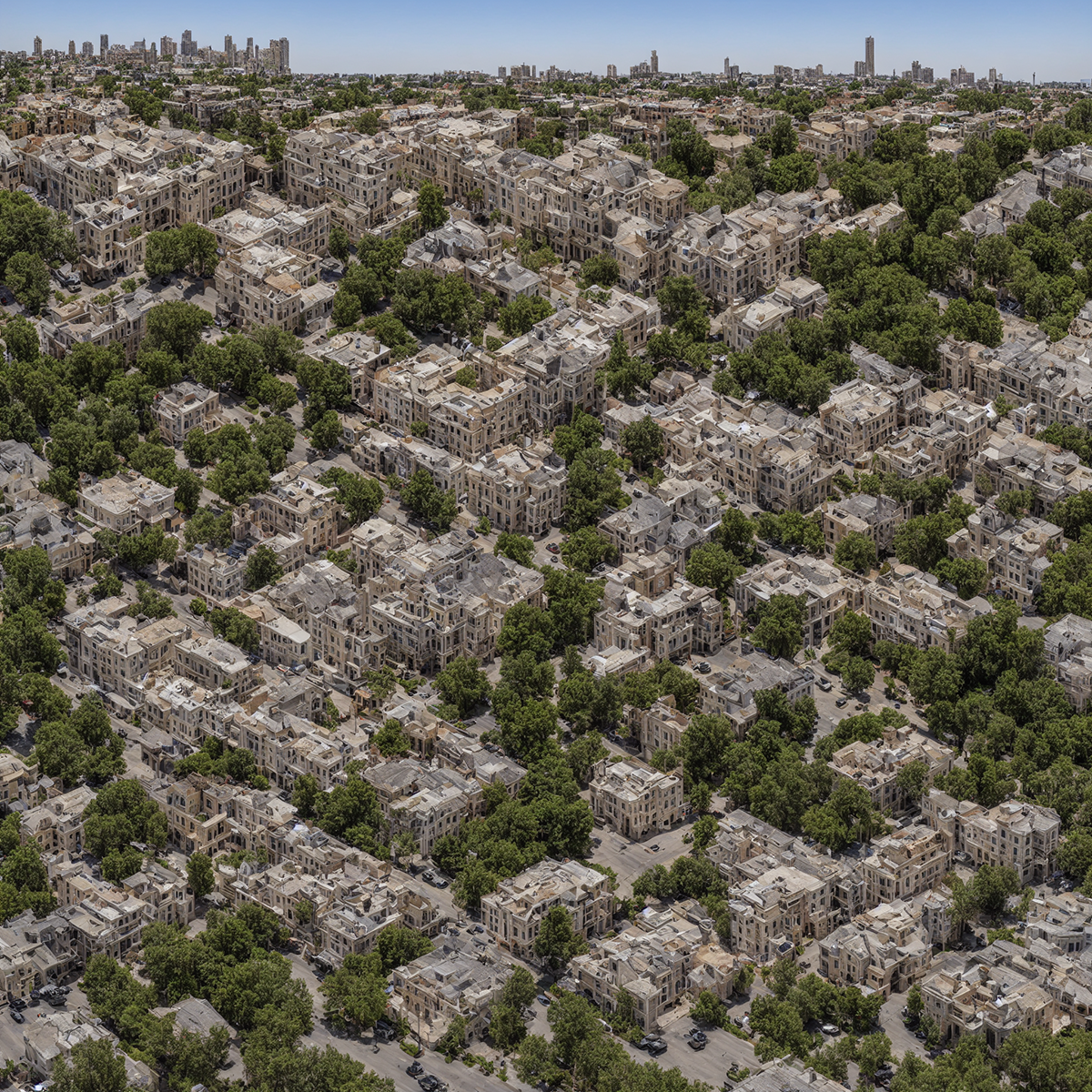

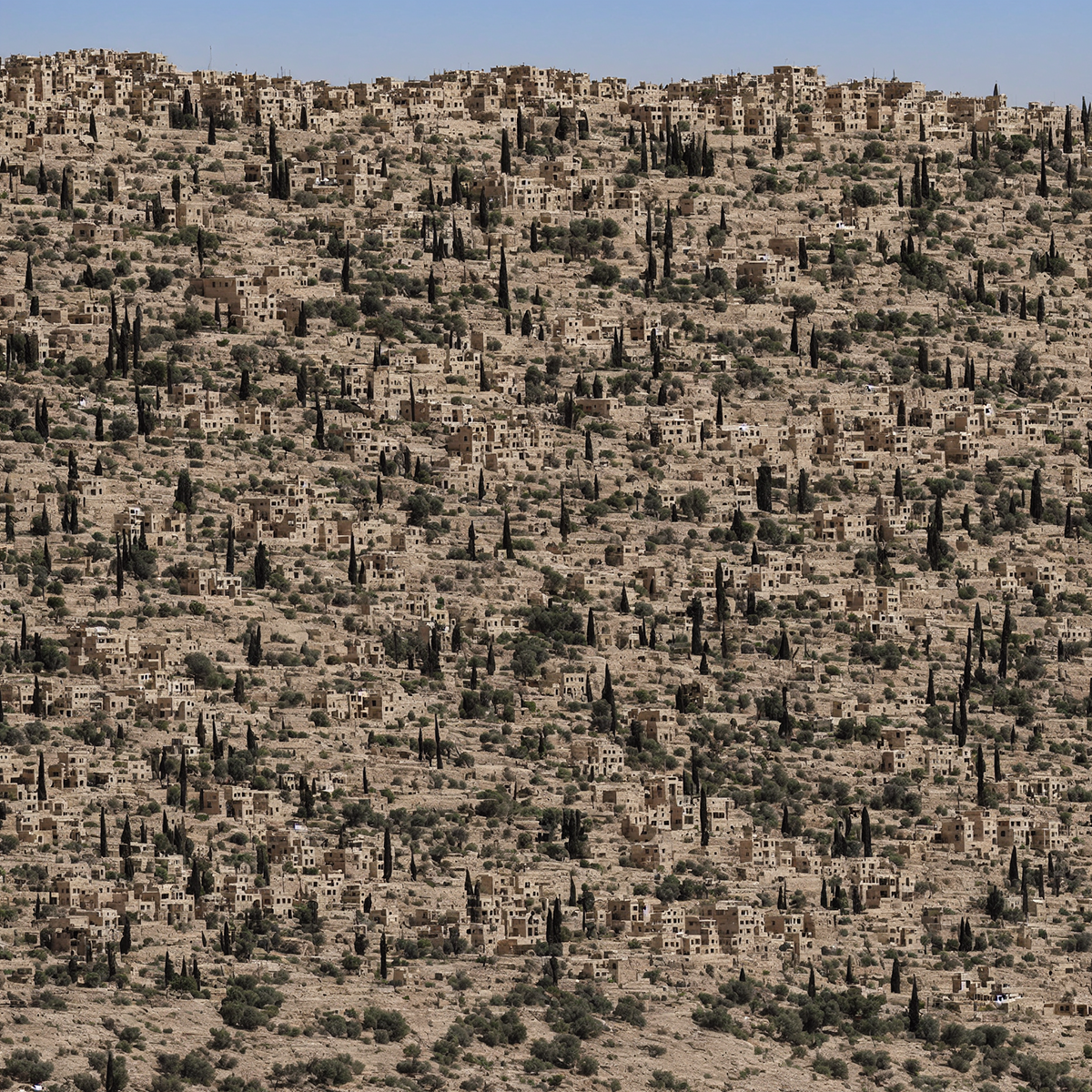


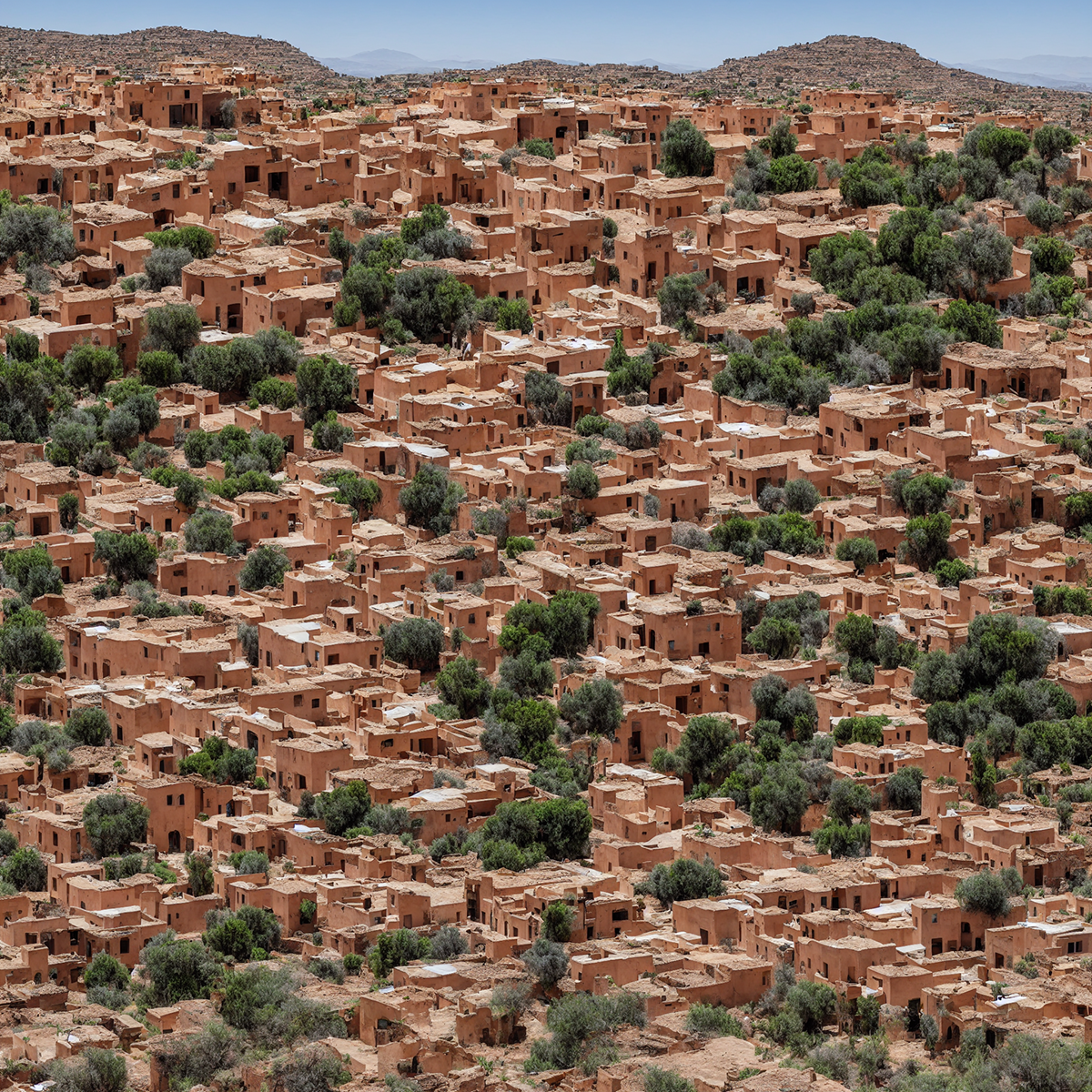
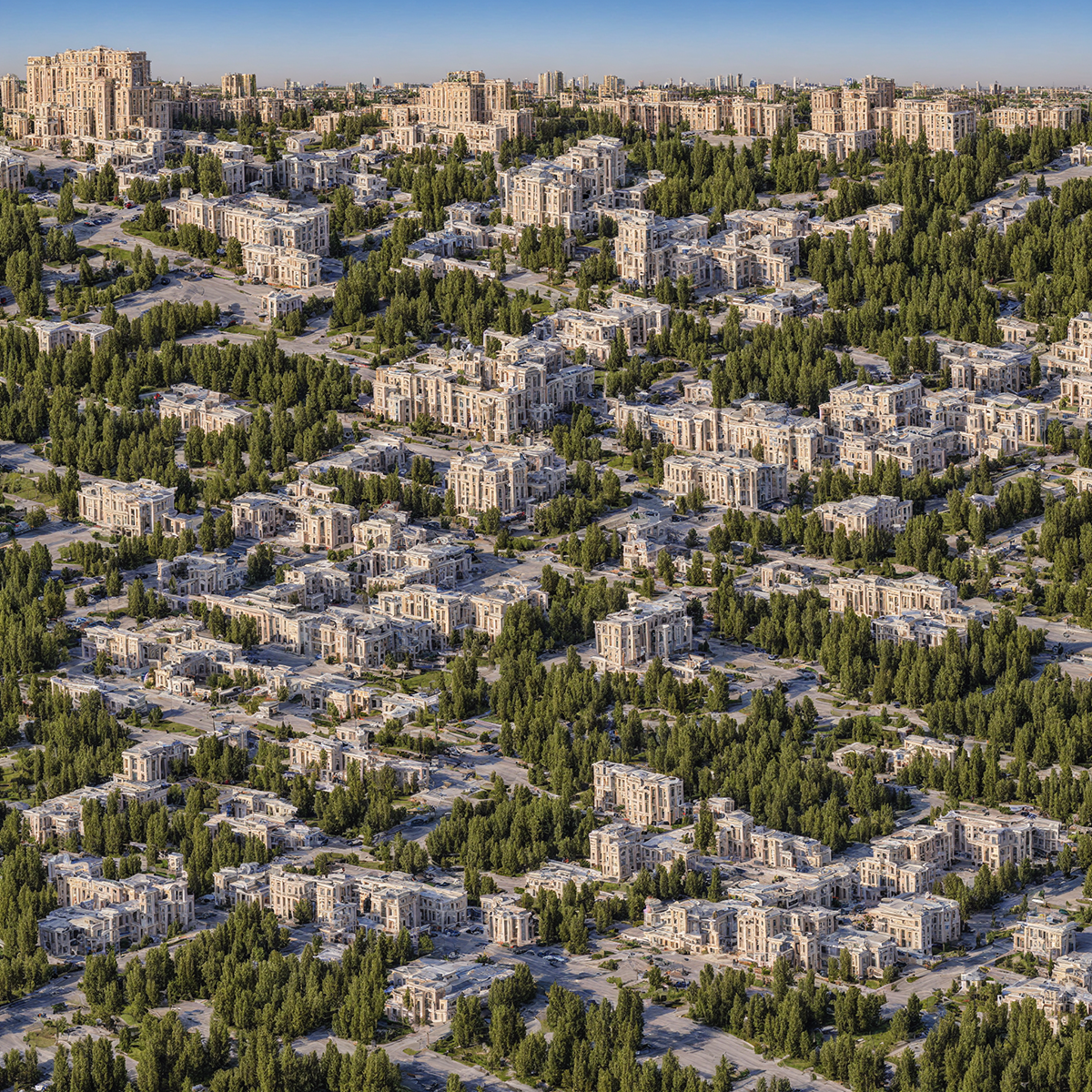
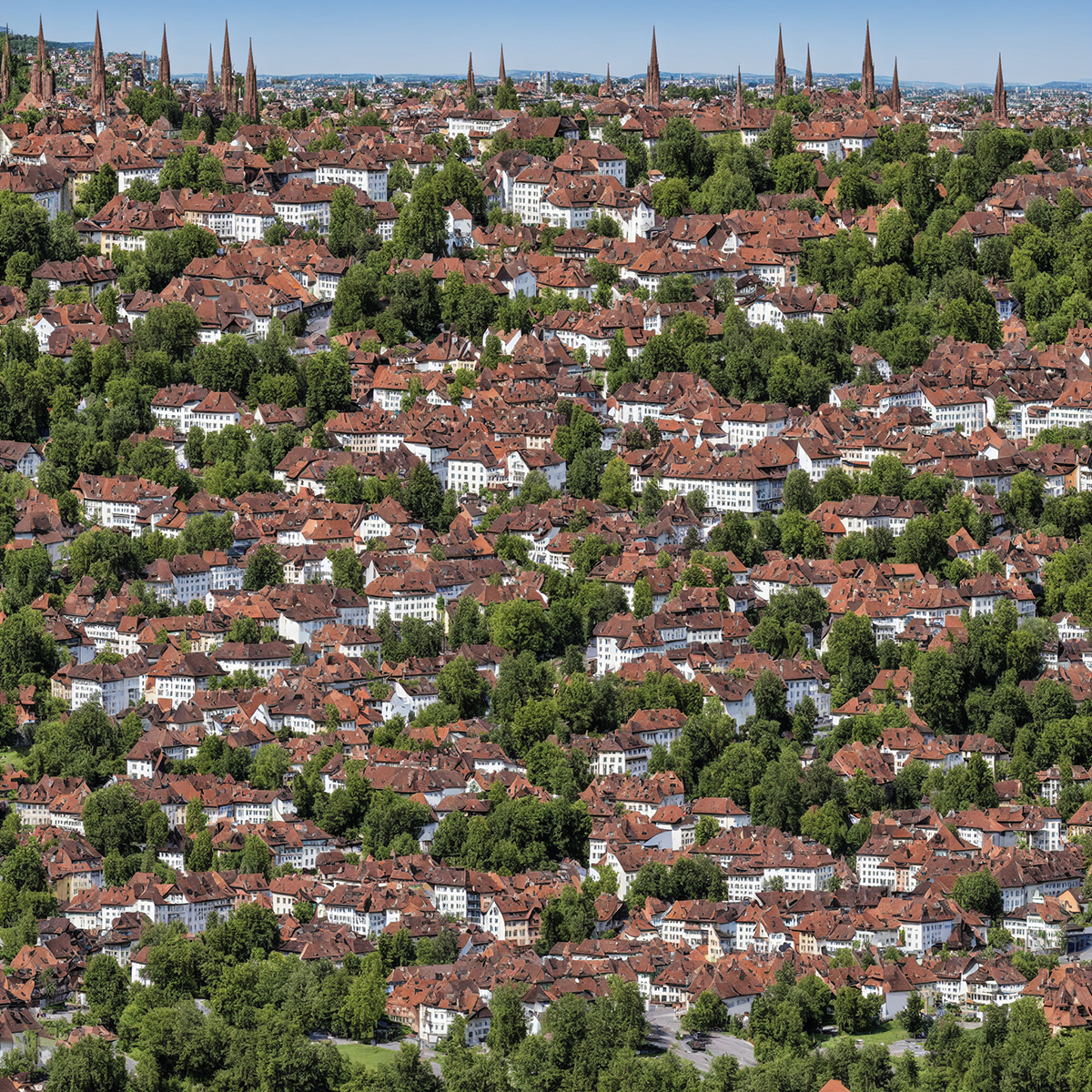
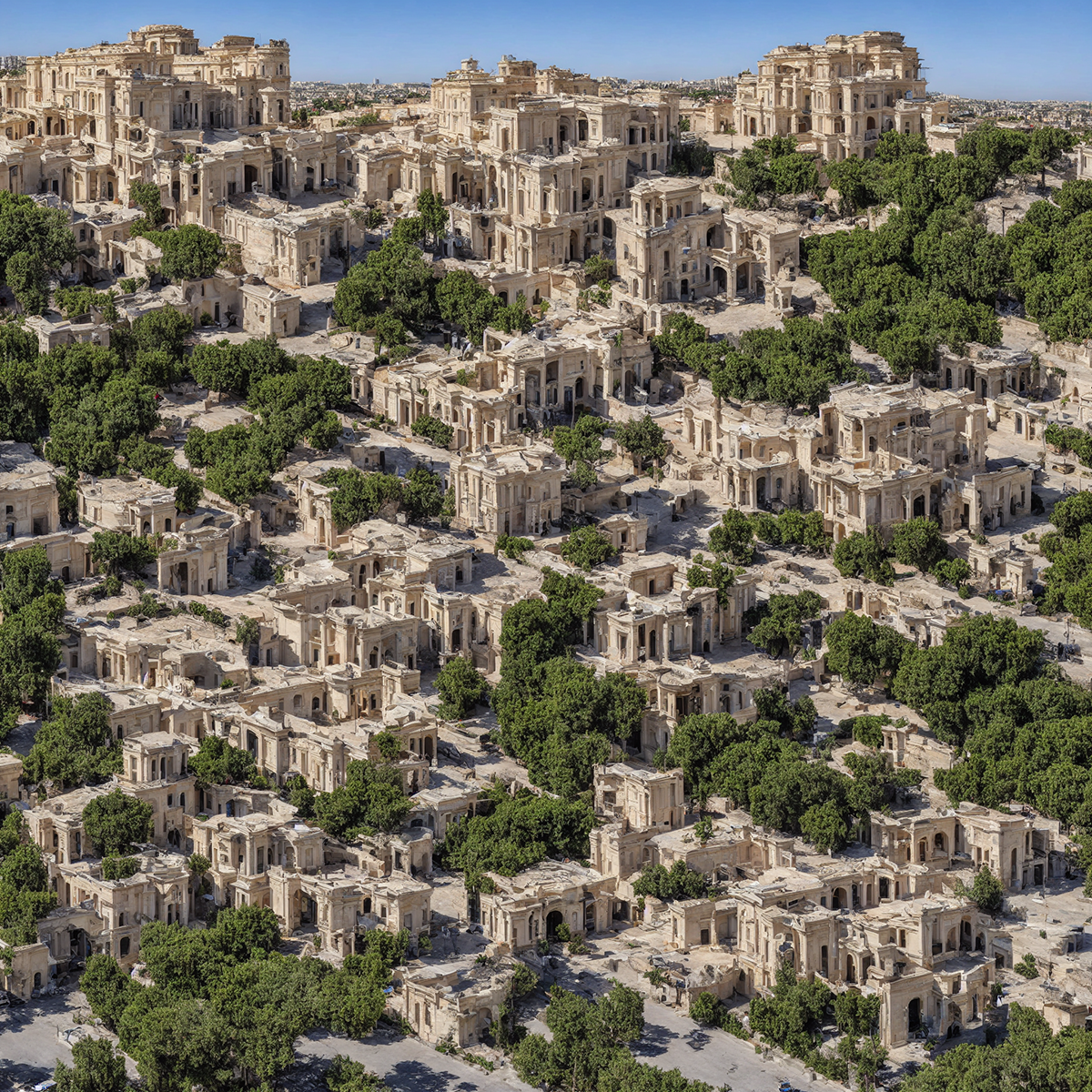
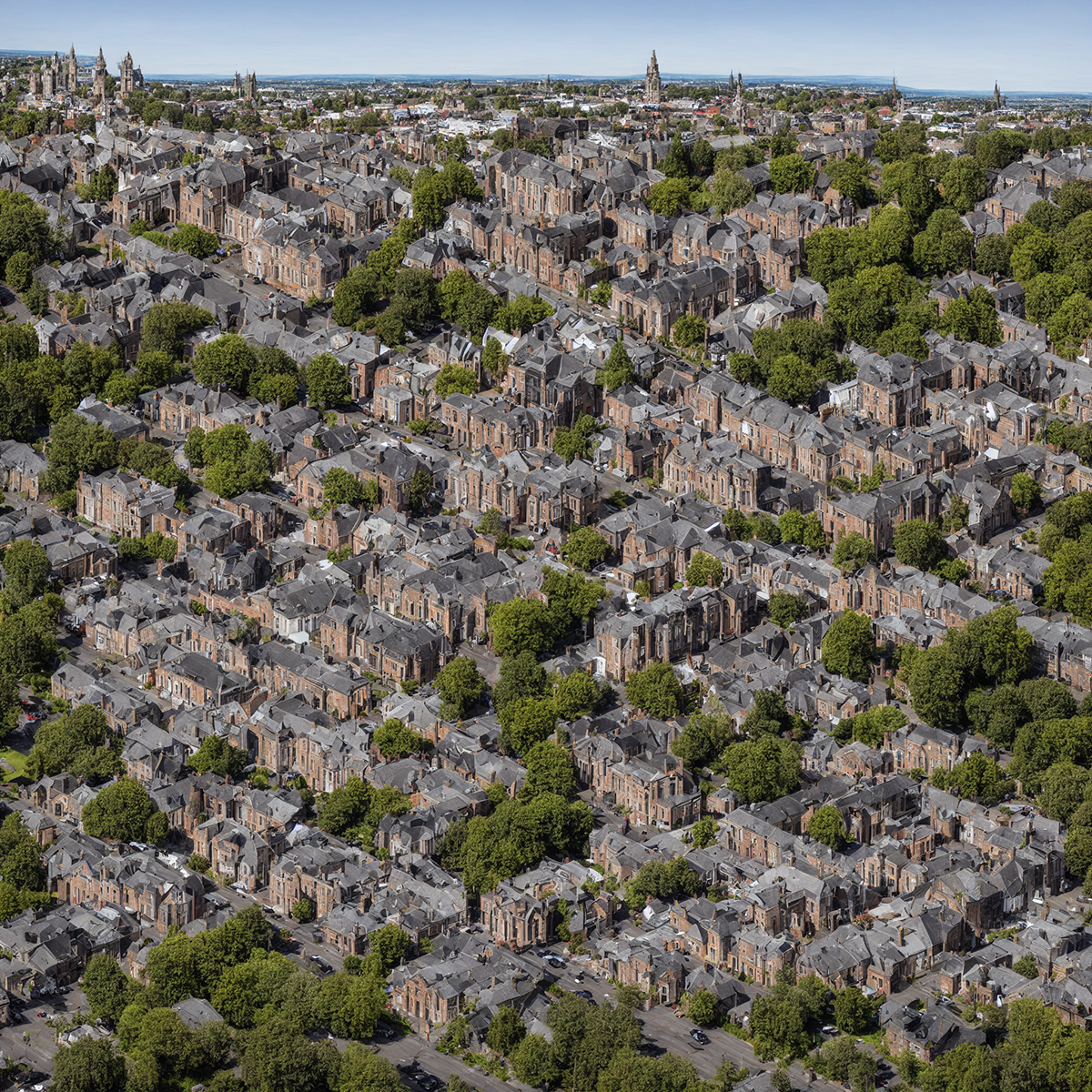
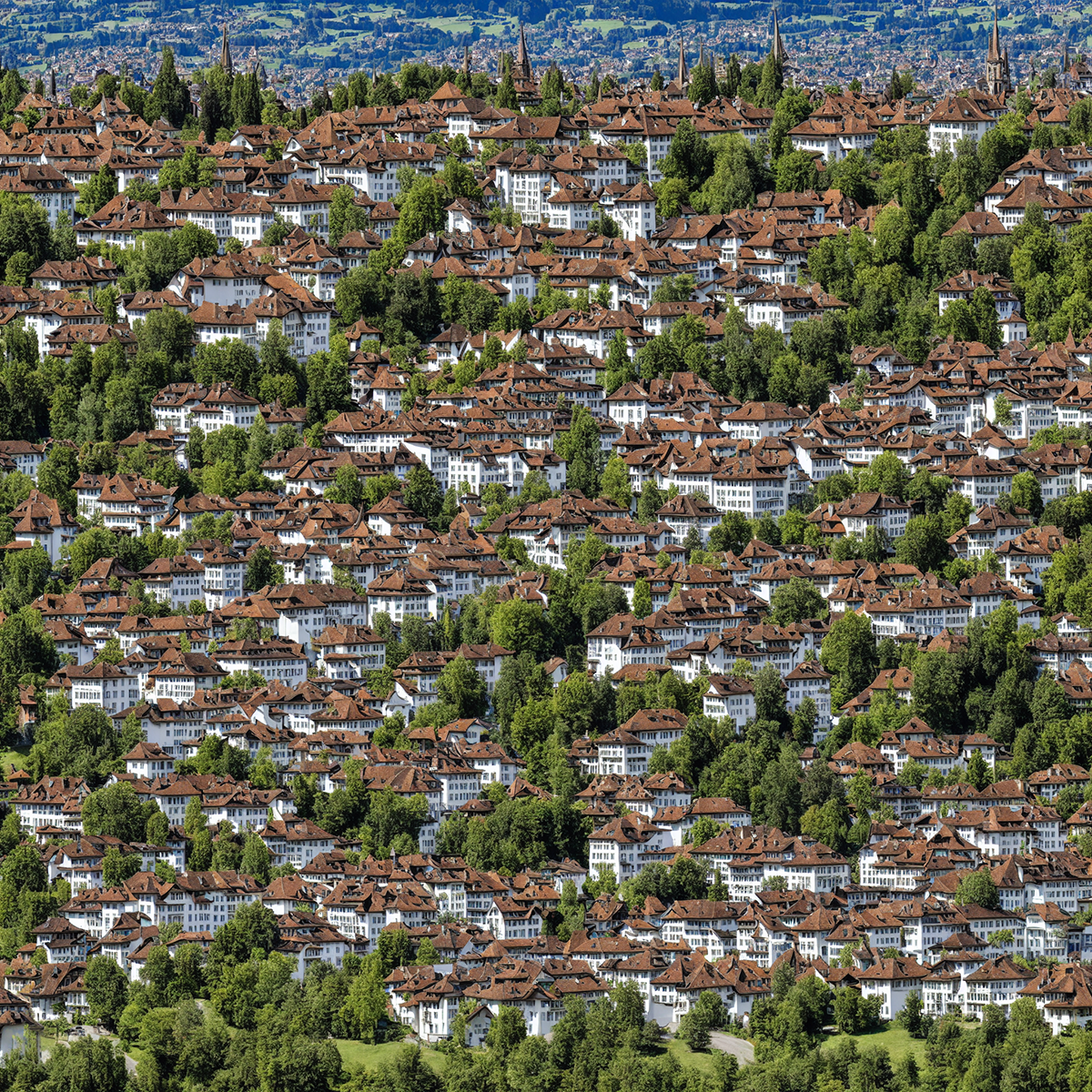